1. Introduction
“Clustering isn’t just an algorithm—it’s a way of seeing patterns the human eye would never catch.”
When I first got into clustering, I thought, “How hard can it be? Just group similar things together.” But once you start working with real-world data, you quickly realize that clustering is both an art and a science. There’s no magic number for clusters, and the same dataset can yield wildly different results depending on the approach you take.
Among all clustering techniques, K-Means has always been my go-to. It’s fast, it’s simple, and when applied correctly, it delivers surprisingly good results. You’ll find it everywhere—from segmenting customers in marketing to compressing images in computer vision.
But here’s the catch: K-Means isn’t perfect. It struggles with complex, non-spherical clusters and can get stuck in local minima. That’s something I learned the hard way when I applied it to high-dimensional text data, only to realize that cosine similarity might have been a better choice.
So why is K-Means still a favorite in data science? Because when you understand its strengths and limitations, you can tweak it, optimize it, and make it work like a charm. Whether you’re using it for anomaly detection, feature learning, or image segmentation, the key is knowing how and when to use it—which is exactly what we’ll cover in this guide.
2. How K-Means Clustering Works: A Deep Dive
I remember the first time I used K-Means on a messy dataset — I thought I had nailed it. The clusters looked neat, the inertia score was low, and everything seemed perfect. But when I visualized the results, I realized I’d grouped data points that had no meaningful connection. That’s when I learned that understanding the mechanics of K-Means is just as important as running the code.
Mathematical Foundation — The Heart of K-Means
At its core, K-Means is all about minimizing one thing: the sum of squared distances between points and their assigned centroids.
Here’s the objective function:

It’s simple in theory — group points around a central point (centroid) to minimize the spread. But in practice, things get tricky.
I’ve found that understanding this function makes a huge difference when things go wrong. For example, if your centroids keep bouncing around or clusters overlap, that’s usually a sign that your data isn’t well-suited for Euclidean distance — a mistake I’ve made more than once.
Convergence Criteria — When to Stop
K-Means stops when either:
✅ Centroids no longer shift significantly.
✅ A maximum number of iterations is reached.
I’ve seen both conditions fail in practice. Sometimes the algorithm stops early even when better clusters are possible. Other times, it keeps looping endlessly with no meaningful improvement. That’s why I always track the inertia score and centroid movement threshold — they’ve saved me from countless wasted hours.
Complexity Analysis — Why K-Means is Fast (but Sometimes Painful)
K-Means has a time complexity of O(n × k × d × i), where:
- n = number of data points
- k = number of clusters
- d = number of features (dimensions)
- i = number of iterations
In simpler terms: More points, more dimensions, and more clusters mean longer processing time.
I remember working on a high-dimensional dataset once — 10,000+ features — and K-Means dragged for ages. Reducing the dimensions using PCA before clustering cut my runtime by nearly half. If you’re dealing with large data, that trick can save you a ton of time.
Step-by-Step Execution with Insights
Here’s where things get interesting — and where experience makes all the difference.
1. Initialization Strategies — The Make-or-Break Step
I can’t stress this enough: Poor initialization ruins everything.
- Random Initialization: Quick, but risky. If you’ve ever run K-Means multiple times and gotten different results each time, this is likely the culprit.
- K-Means++ (My Personal Favorite): This method smartly picks initial centroids that are far apart, drastically improving stability. Since switching to K-Means++, I’ve seen far fewer issues with bad clusters.
Pro Tip: If your clusters keep overlapping or shifting unpredictably, try running K-Means multiple times with different random seeds — it’s a quick way to spot unstable patterns.
2. Cluster Assignment — Why Distance Metrics Matter
By default, K-Means uses Euclidean distance, but I’ve learned that’s not always the best choice.
- Euclidean Distance: Great for compact, spherical clusters.
- Cosine Similarity: Works better for text or high-dimensional data.
- Mahalanobis Distance: Ideal when your features are correlated.
I once applied K-Means to customer data, and the clusters didn’t make sense — some obvious spending patterns were grouped incorrectly. Switching to Cosine Similarity instantly improved the results.
3. Centroid Update and Iterations
Once points are assigned to clusters, K-Means recalculates each centroid by averaging the points in the cluster.
This step feels straightforward, but I’ve seen cases where noisy data throws centroids off. In those cases, I’ve had better luck cleaning outliers before clustering — it often improves results dramatically.
4. Stopping Criteria — When to Call It Quits
In my experience, relying on max iterations alone is risky. Sometimes clusters settle quickly, other times they keep wobbling.
I prefer to combine:
✅ Inertia threshold (stop when the improvement is marginal).
✅ Centroid movement threshold (stop when centroids barely shift).
This combo has saved me from overfitting or burning through unnecessary compute cycles.
5. Local Minima — The Sneaky Trap
Here’s something that caught me off guard early in my career: K-Means can get stuck in a local minimum.
I once ran K-Means on some sales data, and no matter what I tried, the clusters wouldn’t improve. It turned out my initial centroids were unlucky, trapping the algorithm in a bad solution. Running the algorithm multiple times with different initializations solved the problem.
Pro Tip: If you suspect you’re stuck in a local minimum, rerun K-Means with different seeds or switch to K-Means++. It’s a game-changer.
3. Choosing the Right ‘K’: The Hardest Part
“The hardest thing in K-Means? It’s not writing the code—it’s picking the right number of clusters.”
Early on, I made the mistake of assuming there’s a ‘correct’ K for every dataset. Reality check? There isn’t. Some datasets have well-defined natural clusters, while others don’t. I’ve worked on problems where choosing K=3K = 3K=3 gave crystal-clear groupings, and other times where even K=10K = 10K=10 still felt arbitrary. The trick is understanding when to trust the math and when to trust intuition.
Let’s go over the methods I’ve used—and where they shine (or fail).
1. The Elbow Method — Simple, But Not Always Reliable
The Elbow Method is the first thing most people learn when choosing KKK, and for good reason—it’s quick, intuitive, and easy to visualize.
Here’s how it works:
- Run K-Means for multiple values of KKK (e.g., 2 to 10).
- Plot the inertia (sum of squared distances from centroids) against KKK.
- Look for the ‘elbow’—the point where reducing KKK no longer significantly decreases inertia.
📌 When it works well: When clusters are compact and well-separated.
⚠️ Where it fails: When clusters have varying densities or overlap significantly.
Python implementation:
import numpy as np
import matplotlib.pyplot as plt
from sklearn.cluster import KMeans
inertia = []
K_range = range(2, 11)
for k in K_range:
kmeans = KMeans(n_clusters=k, random_state=42)
kmeans.fit(data)
inertia.append(kmeans.inertia_)
plt.plot(K_range, inertia, marker='o')
plt.xlabel('Number of clusters (K)')
plt.ylabel('Inertia')
plt.title('Elbow Method for Optimal K')
plt.show()
I’ve used this method countless times, and when the elbow is obvious, it’s great. But if the graph looks like a smooth curve with no clear elbow? Time to try something else.
2. The Silhouette Score — My Go-To When Elbow Fails
If you’ve ever looked at an Elbow Method plot and thought, “Uhh… I don’t see an elbow at all”, the Silhouette Score is your friend.
It measures how well each point fits into its assigned cluster versus how close it is to the next nearest cluster.

Where:
- a(i)a(i)a(i) = average distance from the point to others in its cluster
- b(i)b(i)b(i) = average distance from the point to the nearest neighboring cluster
Score range:
✅ Closer to +1: Points are well clustered.
❌ Closer to 0: Points are on cluster boundaries.
🚨 Negative: Wrong clustering (points belong elsewhere).
Python Implementation:
from sklearn.metrics import silhouette_score
best_k = 2
best_score = -1
for k in range(2, 11):
kmeans = KMeans(n_clusters=k, random_state=42).fit(data)
score = silhouette_score(data, kmeans.labels_)
if score > best_score:
best_k, best_score = k, score
print(f"Optimal K: {best_k}, Silhouette Score: {best_score}")
📌 When to use it: When clusters are non-uniform in size or density.
⚠️ Where it struggles: Very high-dimensional data, since distances can become meaningless.
In my experience, if the Silhouette Score and Elbow Method give conflicting answers, trust Silhouette—it’s a more direct measure of clustering quality.
3. The Gap Statistic — When You Need Mathematical Rigor
If I’m dealing with high-dimensional data or non-traditional clustering problems, I go with the Gap Statistic.
Unlike the other methods, this one compares the clustering structure of your data against a random distribution. It calculates:

Where:
- WkW_kWk = within-cluster dispersion
- E[logWk]\mathbb{E}[\log W_k]E[logWk] = expected dispersion if data was randomly distributed
Essentially, it tells you how much better your clustering is than random noise.
📌 When to use it: High-dimensional datasets where traditional methods struggle.
⚠️ Downside: Computationally expensive—if you’re working with millions of points, this method might be too slow.
Python Implementation (simplified):
from gap_statistic import OptimalK # Install using `pip install gap-stat`
optimal_k = OptimalK(parallel_backend='joblib')
n_clusters = optimal_k(data, cluster_array=np.arange(2, 11))
print(f"Optimal K: {n_clusters}")
I’ve used this for high-dimensional customer segmentation where Elbow and Silhouette gave misleading results. It’s not always needed, but when you have complex data, this is the most reliable method.
4. Domain Knowledge — The Expert’s Shortcut
Here’s something that no mathematical method can replace: Experience.
Sometimes, you just know how many clusters make sense. If I’m segmenting customers, I might start with:
- 3 clusters: Low, Medium, High spenders.
- 5 clusters: Based on business tiers (Freemium, Basic, Pro, Enterprise, VIP).
If I’m clustering image colors, I might pick:
- K = 8-16 for simple color quantization.
- K = 3-5 for medical image segmentation.
At the end of the day, business logic and real-world knowledge often override ‘optimal’ K values.
4. K-Means for Image Processing: Beyond Theory
“A picture is worth a thousand words, but sometimes, a thousand colors are just too much.”
I remember the first time I used K-Means for image segmentation—I was trying to separate objects in an image, and at first, I thought, “This should be easy.” But as I quickly learned, K-Means works well, but only if you know its quirks.
Let’s go beyond the textbook definition and look at where K-Means truly shines in computer vision—and where it struggles.
Why K-Means is Widely Used in Computer Vision
If you’ve ever worked with images, you know they’re just matrices of pixel values. The challenge? Raw pixels carry too much unnecessary detail. K-Means helps by simplifying an image into meaningful clusters.
Here’s where I’ve personally seen it work best:
1. Color Quantization: Reducing Image Complexity Without Losing Detail
Ever noticed how JPEG compression makes images look blocky? That’s a crude form of color quantization—reducing the number of colors while keeping the visual impact.
K-Means does this way better by clustering similar colors together and replacing them with their centroid. The result?
✅ Smaller file size
✅ Visually appealing images with fewer colors
✅ Faster processing for computer vision tasks
Python Implementation for Color Quantization:
import cv2
import numpy as np
from sklearn.cluster import KMeans
image = cv2.imread('image.jpg')
image = cv2.cvtColor(image, cv2.COLOR_BGR2RGB)
pixels = image.reshape(-1, 3)
kmeans = KMeans(n_clusters=8, random_state=42).fit(pixels)
new_colors = kmeans.cluster_centers_.astype(int)[kmeans.labels_]
quantized_image = new_colors.reshape(image.shape)
cv2.imwrite('quantized_image.jpg', quantized_image)
📌 When it works well: When reducing colors for stylized images, graphics, or compression.
⚠️ Where it struggles: Complex images with gradients—K-Means doesn’t handle smooth transitions well.
2. Image Segmentation: Separating Objects in a Scene
This is where things get exciting. I’ve used K-Means to break down images into regions of interest, whether for object detection or medical imaging.
The idea? Instead of clustering pixel colors, cluster pixel intensities or spatial information. This helps in:
- Medical imaging (separating tumors from normal tissue)
- Satellite imagery (classifying land, water, and vegetation)
- Object detection (background vs. foreground separation)
💡 Pro tip: Instead of using RGB, try HSV or LAB color spaces—they work better for human perception.
Case Study: K-Means for Image Segmentation
I once worked on a project where we needed to segment different materials in a scanned document. The challenge? Shadows and lighting variations made traditional thresholding useless. K-Means saved the day.
Here’s how I did it:
Step 1: Convert Image to a Feature Space
Instead of using raw RGB values, I converted the image into LAB space (better for clustering).
image = cv2.imread('image.jpg')
lab_image = cv2.cvtColor(image, cv2.COLOR_BGR2LAB)
pixels = lab_image.reshape(-1, 3)
Step 2: Apply K-Means on Pixel Values
kmeans = KMeans(n_clusters=3, random_state=42).fit(pixels)
segmented_pixels = kmeans.cluster_centers_.astype(int)[kmeans.labels_]
segmented_image = segmented_pixels.reshape(image.shape)
Step 3: Reconstruct the Segmented Image
cv2.imwrite('segmented_image.jpg', segmented_image)
🔹 Why this worked: LAB space separates brightness from color, so K-Means focused on the real material differences rather than just colors.
Pitfalls of Using K-Means for Image Processing
While K-Means is a great tool, it’s not perfect—and I’ve run into these issues more than once:
- Over-segmentation: Too many clusters can create artificial boundaries.
- ✅ Fix: Choose K wisely (use the Elbow Method or Silhouette Score).
- Under-segmentation: Objects blend together if K is too small.
- ✅ Fix: Use domain knowledge to guide K selection.
- Computational Cost: High-resolution images take forever to cluster.
- ✅ Fix: Downsample the image before clustering, then upscale later.
K-Means vs. Other Clustering Methods in Image Segmentation
I’ve tried different clustering methods for image processing, and here’s how K-Means stacks up:
Algorithm | Strengths | Weaknesses |
---|---|---|
K-Means | Fast, simple, works well for color-based segmentation. | Sensitive to initial centroids, assumes spherical clusters. |
Mean-Shift | No need to predefine K, works well for dense regions. | Computationally expensive. |
DBSCAN | Finds arbitrarily shaped clusters, handles noise well. | Doesn’t work well for color-based segmentation. |
When to use K-Means:
✅ When you need fast, general-purpose image clustering.
❌ When your clusters have complex shapes or overlapping regions (DBSCAN might be better).
5. K-Means for Data Analysis: Real-World Use Cases
“Data is just numbers until you make sense of it. That’s where clustering comes in.”
I’ve personally used K-Means in multiple real-world scenarios, and every time, I’ve learned something new about its strengths and limitations. Some of the most practical applications I’ve worked on involve customer segmentation, fraud detection, and text clustering.
Here’s how K-Means actually works when applied to real business problems.
Customer Segmentation: Understanding Your Users
Let’s say you run an e-commerce platform, and you want to understand your customers. You have thousands—maybe millions—of users, each behaving differently. Some are big spenders, some only buy during discounts, and others browse a lot but rarely purchase.
The question is: How do you segment them efficiently?
That’s exactly where I’ve used K-Means. Instead of treating every customer the same, you can cluster them into meaningful groups based on:
✅ Spending behavior
✅ Purchase frequency
✅ Demographics (age, location, etc.)
✅ Preferred product categories
Here’s a real-world example I worked on:
K-Means for E-Commerce Customer Segmentation
Step 1: Prepare the Data
We extracted historical purchase data and structured it into meaningful features:
- Total amount spent per customer
- Number of purchases
- Average order value
- Days since last purchase
Step 2: Apply K-Means
import pandas as pd
from sklearn.cluster import KMeans
from sklearn.preprocessing import StandardScaler
# Load dataset
data = pd.read_csv('customer_data.csv')
# Selecting features for clustering
X = data[['total_spent', 'num_purchases', 'avg_order_value', 'days_since_last_purchase']]
# Standardizing data (K-Means is sensitive to scale)
scaler = StandardScaler()
X_scaled = scaler.fit_transform(X)
# Applying K-Means
kmeans = KMeans(n_clusters=4, random_state=42)
data['cluster'] = kmeans.fit_predict(X_scaled)
Step 3: Interpret the Results
We ended up with four clear customer segments:
- High-value loyal customers – Spend a lot and return frequently.
- Discount seekers – Only buy during sales.
- One-time buyers – Made one purchase and never returned.
- Inactive users – Haven’t made a purchase in a long time.
🎯 How this helped the business:
- Targeted marketing: Personalized emails for each group.
- Better retention strategies: Special discounts for inactive users.
- Increased revenue: High-value customers received loyalty perks.
📌 Key takeaway: K-Means helps businesses act on data, rather than just collecting it.
Anomaly Detection in Finance and Cybersecurity
“Fraudsters don’t follow patterns—but that’s exactly how we catch them.”
Fraud detection was one of the first real-world problems where I saw clustering make an impact. The idea is simple:
- Normal transactions form clusters (patterns of typical behavior).
- Fraudulent transactions stick out as anomalies.
K-Means is not a dedicated anomaly detection algorithm, but I’ve used it to pre-group normal activity before applying anomaly detection techniques.
K-Means for Fraud Detection: Real Use Case
A fintech company I worked with wanted to flag suspicious transactions. We clustered user behaviors based on:
✅ Transaction amount
✅ Frequency of transactions
✅ Time of day transactions occur
✅ Geolocation (IP address tracking)
After applying K-Means, we found a small cluster that contained outliers—transactions occurring at odd hours from different countries with large amounts. These were flagged for further investigation.
🔹 Challenge: K-Means assumes clusters are of similar size, which fraud isn’t. That’s why I combined it with DBSCAN for better anomaly detection.
📌 Lesson learned: Use K-Means to group normal behavior first—then use other methods to detect fraud more accurately.
Text Data Clustering: Why K-Means Struggles (And How to Fix It)
“Raw text and K-Means? A disaster waiting to happen—unless you transform the data first.”
I learned this lesson the hard way. The first time I tried to cluster news articles with K-Means, it completely failed. Why? Because K-Means relies on numerical data, and raw text is just words.
Here’s the workaround that actually works:
- Convert text into numerical form using TF-IDF (Term Frequency-Inverse Document Frequency).
- Reduce dimensionality with PCA (Principal Component Analysis) to avoid sparse data.
- Apply K-Means on the transformed data.
Example: Clustering News Articles by Topic
Step 1: Convert Text to Vectors (TF-IDF)
from sklearn.feature_extraction.text import TfidfVectorizer
# Sample dataset: News articles
documents = ["Stock markets rise after Federal Reserve speech.",
"New AI model outperforms humans in image recognition.",
"Tesla announces new electric truck with longer battery life."]
vectorizer = TfidfVectorizer(stop_words='english')
X = vectorizer.fit_transform(documents)
Step 2: Reduce Dimensionality with PCA
from sklearn.decomposition import PCA
pca = PCA(n_components=2)
X_pca = pca.fit_transform(X.toarray())
Step 3: Apply K-Means
kmeans = KMeans(n_clusters=3, random_state=42)
labels = kmeans.fit_predict(X_pca)
🎯 Outcome: News articles automatically grouped into:
- Finance (Stock markets)
- AI (Machine learning models)
- Automotive (Electric vehicles)
📌 Key takeaway: K-Means only works for text data if you preprocess it properly.
6. Hands-on Implementation in Python: Bringing K-Means to Life
“Theory is nice, but real understanding comes from rolling up your sleeves and coding.”
When I first started using K-Means in Python, I realized that implementation isn’t always as straightforward as the theory makes it seem. There were moments when my clusters made no sense at all—overlapping points, poor convergence, and frustrating performance issues. Over time, I’ve learned a few practical techniques that can save you hours of debugging.
Let me walk you through a hands-on implementation, focusing on best practices and lessons I’ve learned along the way.
Choosing the Right Dataset: Start Simple, Then Scale
I always recommend starting with a dataset that’s easy to visualize. One of my go-to choices for practicing clustering is the MNIST dataset for image clustering. It’s clear, well-structured, and perfect for seeing how K-Means behaves.
For business use cases, I’ve often worked with e-commerce data for customer segmentation—it’s messy enough to mimic real-world challenges but still manageable in size.
Loading MNIST for Image Clustering
from sklearn.datasets import fetch_openml
import matplotlib.pyplot as plt
# Load MNIST dataset
mnist = fetch_openml('mnist_784', version=1)
X = mnist.data / 255.0 # Normalize pixel values
# Visualizing sample images
plt.figure(figsize=(8, 4))
for i in range(6):
plt.subplot(2, 3, i + 1)
plt.imshow(X.iloc[i].values.reshape(28, 28), cmap='gray')
plt.axis('off')
plt.show()
Implementing K-Means: Tips That Helped Me Avoid Pitfalls
When I first applied K-Means, I underestimated how much data scaling mattered. Without scaling, my results were wildly inaccurate.
Step 1: Scaling the Data
K-Means is heavily influenced by feature magnitudes, so scaling is a must. I’ve had the best results using StandardScaler for numerical data and MinMaxScaler for pixel values or images.
from sklearn.preprocessing import StandardScaler
from sklearn.cluster import KMeans
# Standardizing features
scaler = StandardScaler()
X_scaled = scaler.fit_transform(X)
# Applying K-Means
kmeans = KMeans(n_clusters=10, random_state=42)
labels = kmeans.fit_predict(X_scaled)
Step 2: Visualizing Results
Whenever possible, I visualize my clusters—especially when testing different initialization strategies or cluster counts.
Improving K-Means with PCA: A Trick That Changed Everything
In one of my earlier projects, I struggled with a dataset that had hundreds of features. K-Means kept producing unstable clusters, and I couldn’t figure out why.
That’s when I realized PCA could help. By reducing the dataset’s dimensions before applying K-Means, I improved both speed and accuracy dramatically.
PCA + K-Means Implementation
from sklearn.decomposition import PCA
# Reduce to 50 dimensions before clustering
pca = PCA(n_components=50)
X_pca = pca.fit_transform(X_scaled)
# Apply K-Means on PCA-reduced data
kmeans = KMeans(n_clusters=10, random_state=42)
labels = kmeans.fit_predict(X_pca)
🔹 Pro Tip: In my experience, PCA typically works best when you choose enough components to retain 90–95% of the dataset’s variance.
Evaluating Clustering Quality: What Metrics Really Matter
“If you can’t measure it, you can’t improve it.”
I’ve tried several evaluation metrics for clustering, but these three stand out:
✅ Inertia (aka Within-Cluster Sum of Squares)
- Measures how tightly data points are clustered.
- Lower inertia is better, but don’t rely on this alone—it often decreases as you increase clusters.
✅ Silhouette Score
- Measures how well data points fit within their assigned cluster.
- Values range from -1 to 1; higher is better.
✅ Davies-Bouldin Index
- Measures cluster compactness and separation.
- Lower scores indicate better-defined clusters.
Example Code for Evaluating Clusters
from sklearn.metrics import silhouette_score, davies_bouldin_score
print(f"Inertia: {kmeans.inertia_}")
print(f"Silhouette Score: {silhouette_score(X_pca, labels):.2f}")
print(f"Davies-Bouldin Index: {davies_bouldin_score(X_pca, labels):.2f}")
Personal Tip: I’ve found that a high silhouette score paired with a low Davies-Bouldin index is a solid indicator of good clustering.
Scaling K-Means: When Your Dataset Outgrows Your Machine
If you’ve ever tried running K-Means on millions of data points, you know the frustration—slow convergence, memory overload, or even complete crashes.
I hit this wall myself when clustering a 100M+ row transaction dataset. After experimenting with several optimizations, two solutions stood out:
1. RAPIDS cuML (GPU-Accelerated K-Means)
I can’t stress this enough—if you’re working with large datasets and have access to a GPU, RAPIDS cuML is a game-changer.
It’s incredibly fast and mimics sklearn
syntax, so adapting your code is simple.
import cuml
from cuml.cluster import KMeans as cuKMeans
# GPU-accelerated K-Means
kmeans = cuKMeans(n_clusters=10, random_state=42)
labels = kmeans.fit_predict(X_pca)
In one of my projects, RAPIDS reduced clustering time from 45 minutes to just 3 minutes—a massive improvement.
2. Apache Spark’s MLlib (For Distributed Computing)
For even larger datasets (think billions of rows), I’ve had success scaling K-Means with Spark’s MLlib. It’s designed for distributed computing and handles massive data far better than traditional tools.
from pyspark.ml.clustering import KMeans
from pyspark.sql import SparkSession
# Initialize Spark
spark = SparkSession.builder.appName('KMeans').getOrCreate()
# Load data into Spark DataFrame
data = spark.read.csv('large_dataset.csv', header=True, inferSchema=True)
# Train K-Means model
kmeans = KMeans().setK(10).setSeed(42)
model = kmeans.fit(data)
# Assign clusters
result = model.transform(data)
result.show(5)
Final Thoughts: What I Wish I Knew Earlier
If there’s one thing I’ve learned about K-Means, it’s that implementation is rarely perfect on the first try.
✅ Start small, visualize your clusters, and iterate.
✅ Use PCA if your dataset has too many features.
✅ For massive datasets, RAPIDS or Spark can save you hours of computation.
K-Means may seem simple, but mastering it takes practice. The more you experiment, the better you’ll get at recognizing when (and when not) to use it.
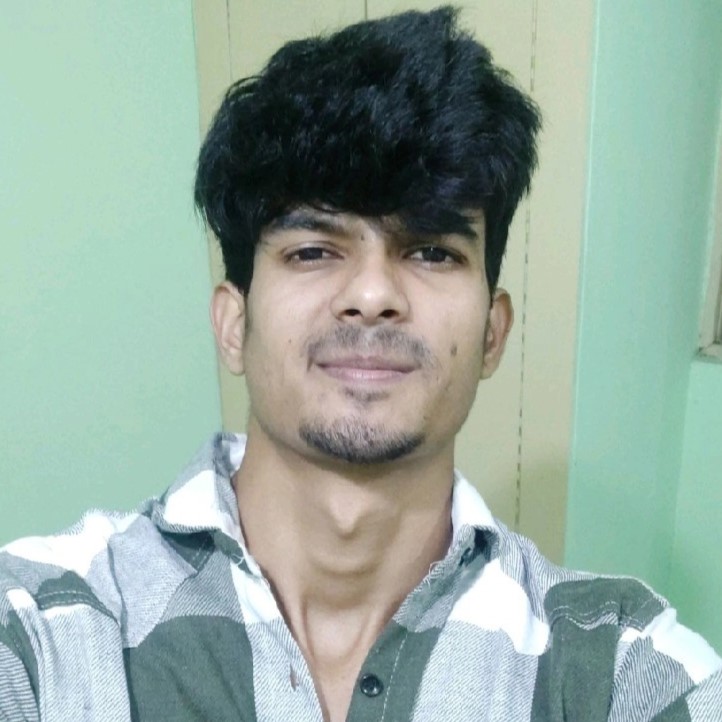
I’m a Data Scientist.