1. Introduction
“Tools don’t make you smart. Using them well does.”
When I first started integrating Langflow into my LLM stack, I wasn’t looking for yet another wrapper around LangChain. I needed something that could let me orchestrate complex chains, inject runtime inputs, and ship prototypes without babysitting JSON configs every five minutes.
Langflow nailed that — but only when paired with a proper API-driven workflow. The UI is great for visualizing flows, sure. But if you’re like me and you’re deploying to production, you want full control from code — not from clicks.
That’s exactly what this guide is about.
You’re going to see how I set up Langflow’s API with a Node.js environment (yes, not Python), execute flows dynamically, inject real-time variables, and handle outputs like a pro. By the end, you won’t just understand how Langflow’s API works — you’ll have code you can drop into your backend today.
Let’s skip the fluff and get straight into it.
2. Pre-Requisites
Here’s what I personally had in place before I even touched the API:
Node.js (v18+ recommended)
I use nvm
to manage versions. If you’re switching between projects often, this saves you from version hell:
nvm install 18
nvm use 18
If you’re using an older version, especially anything pre-v16, Langflow’s API responses might give you trouble with newer ES modules or fetch polyfills — just a heads-up.
Essential npm packages
Run this once:
npm install axios dotenv
axios
is your best bet for interacting with Langflow’s API.dotenv
helps keep your API tokens out of the code — which, from experience, you absolutely want once you start debugging in production.
Langflow Running Locally or on a Remote Host
You’ve got two options:
- Local Setup (for testing and prototyping) I run Langflow locally using Docker. Here’s the minimal setup I used:
git clone https://github.com/logspace-ai/langflow.git
cd langflow
docker-compose up --build
This exposes the Langflow API at http://localhost:7860
by default.
2. Remote Setup (for teams or prod testing) If you’re deploying Langflow to a server (say, an EC2 instance), expose it over a secure tunnel and use HTTPS with proper headers. I usually pair this with Nginx and a simple basic auth if I don’t have OAuth in place yet.
API Token Access
Langflow might not ship with authentication out of the box (yet), but in my case, I still use .env
for clean separation:
LANGFLOW_API_URL=http://localhost:7860
In your Node.js project, make sure you’re loading this early:
require('dotenv').config();
Trust me — this saves you from accidentally hardcoding localhost URLs and wondering why things break in staging.
3. Understanding the Langflow API (Only What You Need)
“I don’t care how it works under the hood unless the hood’s on fire.”
I’ll be honest — when I first looked at the Langflow API, I expected the usual over-engineered chaos. But it’s surprisingly lean. That’s a good thing.
You don’t need to memorize twenty endpoints or dig through abstract specs. In my experience, three endpoints cover 95% of real-world use cases. Here’s the shortlist that I personally use in production:
/build
— Create or Modify a Flow
This endpoint is where I create flows programmatically. Instead of clicking around the Langflow UI, I just POST a flow JSON directly from code.
Here’s a basic payload I’ve used:
{
"data": {
"nodes": {
"PromptTemplate_1": {
"id": "PromptTemplate_1",
"type": "PromptTemplate",
"template": "What's the capital of {country}?",
"input_variables": ["country"]
},
"LLMChain_1": {
"id": "LLMChain_1",
"type": "LLMChain",
"prompt": "PromptTemplate_1"
}
},
"edges": [
{
"source": "PromptTemplate_1",
"target": "LLMChain_1"
}
]
},
"name": "SimpleQuestionFlow"
}
⚠️ Pro tip: I usually export a flow from the Langflow UI once, then modify that exported JSON in code. It’s way faster than crafting it by hand.
/run
— Execute a Flow
This one’s the heart of the operation.
Once I have a flow (either created via /build
or manually built in the UI), I trigger it using /run
. I pass inputs dynamically, depending on the user or context.
Here’s an example I’ve used to run the above flow:
POST /run
Content-Type: application/json
{
"flowId": "f7e2c0d9-xxxx-xxxx-xxxx-bde1c07d25c8",
"inputs": {
"country": "Japan"
}
}
And yes — you’ll get back a structured response with the output from the final node (in this case, LLMChain). It’s usually wrapped in a response object, so I always extract it safely before sending it downstream.
/flows/{id}
— Load, Update, or Delete a Flow
I use this when I want to:
- Load a flow for inspection
- Update parts of it on the fly (like changing prompt templates)
- Or just clean up test flows I don’t need anymore
For example, here’s how I fetch an existing flow:
GET /flows/f7e2c0d9-xxxx-xxxx-xxxx-bde1c07d25c8
You’ll get the full structure back — nodes, edges, metadata. You can patch this using a PATCH
request with partial updates. Just make sure the node structure stays valid.
Bonus: API Docs via Swagger
Langflow ships with a Swagger/OpenAPI interface. I personally use it to test new endpoints quickly without writing any code. If it’s enabled, you can usually hit:
Bonus: API Docs via Swagger
Langflow ships with a Swagger/OpenAPI interface. I personally use it to test new endpoints quickly without writing any code. If it’s enabled, you can usually hit:
It gives you a nice playground to send requests and see schemas. Super useful when you’re debugging a weird input format or checking which field is required.
4. Langflow Project Setup (Real Code, Minimal Setup)
“The best setup is the one you don’t have to explain twice — to yourself.”
I don’t like bloated boilerplates. I prefer working setups that are production-ready without becoming a 14-file maze. Here’s how I personally structured my Langflow + Node.js project. It’s minimal, but battle-tested.
a. Project Structure
Here’s what my folder looks like in one of my actual projects:
📁 langflow-node/
┣ 📄 index.js
┣ 📄 .env
┗ 📁 utils/
┗ 📄 langflowClient.js
Simple. Everything sits in one directory. No build steps. No framework overhead.
b. Code: langflowClient.js
This is where I setup a clean, reusable Axios instance with interceptors. I always do this — even for internal APIs — because the error logging pays off in production.
// utils/langflowClient.js
const axios = require("axios");
const langflowClient = axios.create({
baseURL: process.env.LANGFLOW_API_URL,
timeout: 10000,
headers: {
"Content-Type": "application/json",
},
});
// Error interceptor (optional but super useful)
langflowClient.interceptors.response.use(
(response) => response,
(error) => {
if (error.response) {
console.error("Langflow API Error:", {
status: error.response.status,
data: error.response.data,
});
} else {
console.error("Langflow Client Error:", error.message);
}
return Promise.reject(error);
}
);
module.exports = langflowClient;
You might be wondering: why not just use fetch
?
I did — briefly. But trust me, once you hit error 500s or malformed JSON from a flow, axios
+ interceptors gives you real diagnostics.
c. Code: index.js
Now this is where it gets fun.
I’m loading an existing flow, passing dynamic inputs, and triggering execution — all from code. This is the flow I usually start with during early integration.
// index.js
require("dotenv").config();
const langflowClient = require("./utils/langflowClient");
const FLOW_ID = "f7e2c0d9-xxxx-xxxx-xxxx-bde1c07d25c8"; // Replace with your actual Flow ID
async function runLangflow() {
try {
// Optional: fetch the flow for logging/debug
const { data: flowData } = await langflowClient.get(`/flows/${FLOW_ID}`);
console.log("Loaded Flow:", flowData.name);
// Dynamic input payload
const inputs = {
country: "Canada",
};
// Trigger execution
const response = await langflowClient.post("/run", {
flowId: FLOW_ID,
inputs,
});
const output = response.data?.result || "[No output received]";
console.log("Langflow Output:", output);
} catch (error) {
console.error("Execution failed:", error.message);
}
}
runLangflow();
Here’s the deal:
This tiny script is all I need to validate flows before I plug them into bigger services. I’ve used it inside job queues, Slack bots, even a lightweight Next.js frontend for demos.
This setup has saved me hours. I can spin up a new Langflow-backed Node.js integration in under 5 minutes — and that includes writing the flow itself.
5. Building and Running a Flow Programmatically
“If you can build it by hand, you can automate it in code — and Langflow is no exception.”
I’ll be straight with you: the Langflow UI is great for prototyping. But when you’re building systems at scale — maybe chaining multiple custom agents or dynamically adjusting pipelines — the UI becomes a bottleneck. That’s when the /build
and /run
endpoints start to shine.
Let’s walk through how I create and trigger flows programmatically — directly from Node.js.
a. Code: createFlow()
Here’s a full example of how I’ve created Langflow flows from code.
// createFlow.js
require("dotenv").config();
const langflowClient = require("./utils/langflowClient");
async function createFlow(flowName = "Generated Flow") {
try {
// This structure came from exporting a working flow from the UI
const baseFlow = {
name: flowName,
description: "Programmatically created flow",
data: {
nodes: {
"1": {
id: "1",
type: "PromptTemplate",
template: "What is the capital of {{country}}?",
input_variables: ["country"],
},
"2": {
id: "2",
type: "LLMChain",
inputs: {
prompt: "1",
llm: "openai",
},
},
},
edges: [
{ source: "1", target: "2", sourceHandle: "output", targetHandle: "prompt" },
],
},
};
const response = await langflowClient.post("/build", baseFlow);
console.log("Flow created:", response.data?.id);
return response.data?.id;
} catch (err) {
console.error("Failed to create flow:", err.message);
}
}
module.exports = createFlow;
Pro tip: If you’re ever unsure what the payload should look like, just build a working flow in the Langflow UI, export it, and tweak it in code. That’s exactly how I figured out the JSON structure above.
b. Code: runFlow(flowId, inputs)
Once you’ve created a flow, triggering it is pretty straightforward — but there are quirks in the input structure you’ll want to be aware of.
// runFlow.js
const langflowClient = require("./utils/langflowClient");
async function runFlow(flowId, inputs = {}) {
try {
const response = await langflowClient.post("/run", {
flowId,
inputs,
});
// The output is usually nested — handle it carefully
const result = response.data?.result;
console.log("Flow Output:", result);
return result;
} catch (err) {
console.error("Failed to run flow:", err.message);
}
}
module.exports = runFlow;
This might surprise you:
Inputs aren’t mapped to node IDs. They’re mapped to variable names like country
, which must match the input_variables
defined in the PromptTemplate
. This part tripped me up the first time — especially when dynamically wiring multiple templates.
Real Usage Example
And just to close the loop — here’s how I wired it together in index.js
:
// index.js
const createFlow = require("./createFlow");
const runFlow = require("./runFlow");
(async () => {
const flowId = await createFlow("Auto-Generated Flow");
if (!flowId) return;
const inputs = { country: "Germany" };
const result = await runFlow(flowId, inputs);
console.log("Final Answer:", result);
})();
I’ve used this setup in internal tooling that needed dynamic chain generation based on user input. It’s lightweight, fast, and scalable — exactly what you want in real-world data apps.
6. Advanced Use Case: Dynamic Prompt Injection
“Templates are great — until the real world shows up with its chaos.”
I ran into this while building a chatbot interface that needed to modify prompts on the fly based on incoming user input. Not just variables, but entire prompt structures injected dynamically mid-conversation. And yes, I made it work with Langflow.
The Scenario
Let’s say you’ve got a ChatPromptTemplate
node in your flow. It’s structured around a placeholder like {{user_input}}
. Now you want to feed new values into that dynamically — every single time a user sends a message.
Sounds basic, but here’s the trick: Langflow expects specific variable names tied to your node definitions. You need to map your data directly into those during the /run
.
Code: Dynamic Injection in Practice
// chatBotFlow.js
const runFlow = require("./runFlow");
async function injectPrompt(flowId, userInput) {
try {
const result = await runFlow(flowId, {
user_input: userInput,
});
console.log("Bot says:", result);
return result;
} catch (err) {
console.error("Dynamic injection failed:", err.message);
}
}
Now if your flow has a PromptTemplate
node expecting user_input
, this just works. I’ve used this approach for voice-to-AI chat apps, Slackbot integrations, and even terminal-based agents.
You might be wondering: What if the variable name changes or there are multiple prompt nodes?
I usually export the flow JSON, inspect theinput_variables
, and build a mapping layer in Node.js to keep it clean and scalable.
7. Working with Custom Components
“Langflow gives you a sandbox — custom components let you bring your own tools.”
This part took me a bit of experimentation, but yes — you can integrate custom nodes like Python scripts or specialized logic into Langflow. It’s not officially documented in depth, but here’s how I’ve done it.
The Use Case
I had a case where the LLM needed structured context — something like transforming tabular data into a natural language summary before it hits the LLM. I didn’t want to hardcode this logic into the prompt. So I created a custom Python function as a Langflow node.
Registering a Custom Component
This assumes you’re working with a self-hosted Langflow setup (which you probably are if you’re building anything serious).
In your local Langflow project, you can register a custom component like so:
# my_components/my_custom_node.py
from langflow.interface.custom_component import CustomComponent
class MyDataPreprocessor(CustomComponent):
def __init__(self):
super().__init__()
def build_config(self):
return {
"raw_data": {"type": "string", "required": True}
}
def run(self, raw_data):
# Custom transformation logic
return f"Summarized: {raw_data[:100]}..."
Then register it in Langflow’s component registry and restart the server.
Calling It from Node.js
Once your custom node is part of the flow, treat it like any other node — feed it data, wire it to downstream nodes (like an LLMChain), and trigger it via the API.
// runCustomComponent.js
const runFlow = require("./runFlow");
(async () => {
const inputs = {
raw_data: "User purchase logs for last 7 days: Item A x3, Item B x1...",
};
const result = await runFlow("your-flow-id", inputs);
console.log("Processed Output:", result);
})();
This setup let me run live data through a custom Python chain, transform it, and generate LLM-ready output — all triggered from Node.js. That’s real production orchestration.
8. Deploying This in Production (The Stuff That Saves You at 3 AM)
“Production is where theory goes to die.”
You’ve got your Langflow flow wired up, dynamic inputs flowing, maybe a Slackbot or a user-facing tool on top — now what? You’re not done until you’ve locked it down, logged it out, and made it bulletproof. Here’s what I do in my own deployments:
Security: No Excuses Here
1. Secrets stay out of your codebase. Period.
I never hardcode Langflow API keys or base URLs. I’ve used .env
during dev, but for staging/prod, I personally prefer AWS Secrets Manager or Vault depending on the infra.
# .env (for local dev only)
LANGFLOW_API_KEY=sk-prod-your-key-here
LANGFLOW_API_URL=https://your-instance/api
In langflowClient.js
:
require("dotenv").config();
const axios = require("axios");
const langflowClient = axios.create({
baseURL: process.env.LANGFLOW_API_URL,
headers: {
Authorization: `Bearer ${process.env.LANGFLOW_API_KEY}`,
},
});
2. Rate limiting + retries.
You never want to DDoS your own Langflow server or have flaky behavior on failure. I use axios-retry:
const axiosRetry = require("axios-retry");
axiosRetry(langflowClient, {
retries: 3,
retryDelay: axiosRetry.exponentialDelay,
});
Performance: Don’t Wait for the Bottleneck
Parallel flow execution (when stateless):
I’ve batched Langflow executions using Promise.all()
— especially useful when running multiple flows per user action (e.g., response + summarization).
await Promise.all([
runFlow(flowId, inputA),
runFlow(flowId, inputB),
]);
Cache repeated executions:
Langflow isn’t always cheap, so I use Redis to store flow results if the input signature hasn’t changed. Sometimes, just an in-memory Map()
gets the job done for short-lived caching.
Logging & Observability: Make Debugging Boring
I’ve made the mistake of just console.log()
-ing stuff. Don’t. Use pino
or winston
and log structured JSON. It’s saved me countless hours debugging user prompts gone wrong.
const pino = require("pino");
const logger = pino({ level: "info" });
logger.info({ flowId, input }, "Running Langflow...");
Pro tip: Redact sensitive inputs if you’re logging user data.
9. Conclusion: So What Did We Actually Build?
Let’s take a step back.
Over this guide, you and I built a real-world Node.js integration with Langflow. We skipped the fluffy parts, and instead focused on:
- Programmatically building and running flows using the Langflow API.
- Injecting inputs dynamically — not just for demos, but for real-time user experiences.
- Wiring it all up into a production-ready interface with logging, security, and retries.
- Extending Langflow with custom components and real-time orchestration logic.
Where Langflow + Node.js Shines
In my experience, this combo works incredibly well for:
- Slackbots, internal copilots, and live chat interfaces.
- Data ops pipelines, especially when enriching or summarizing.
- Integrating LLMs into Express.js APIs with a controlled, modular backend.
- Rapid iteration — change your flow in the UI, push inputs via Node.js, ship fast.
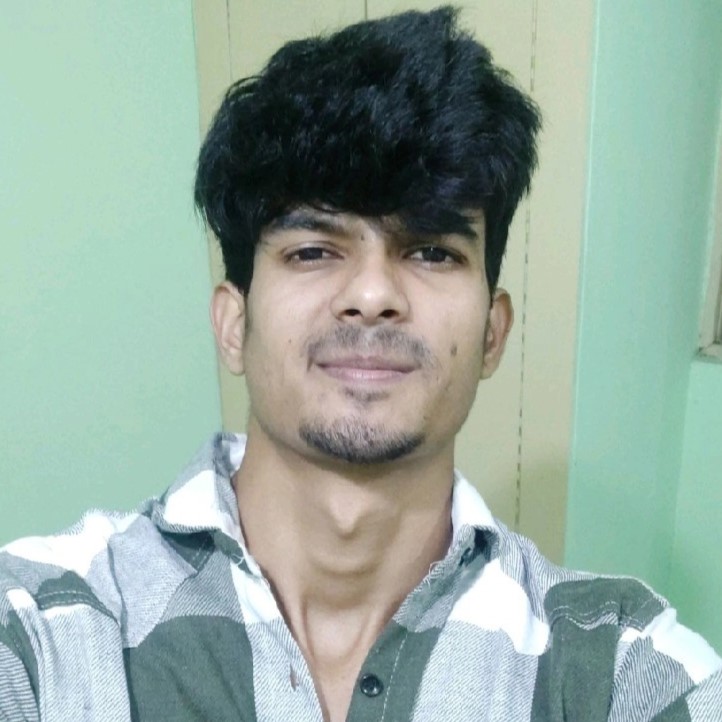
I’m a Data Scientist.