1. Intro: Why Fine-Tune LLaMA Instead of Starting from Scratch?
“Give me a GPU and a foundation model, and I’ll show you results that a scratch-built model could only dream of.”
When I first started working with LLaMA, I wasn’t aiming to reinvent anything. I just needed a model that could adapt quickly to my domain-specific tasks—stuff like customer support summarization and internal knowledge base Q&A. Training from scratch?
Way too expensive and not worth the hassle when you already have a beast like LLaMA available.
I’ve personally had the best results with LLaMA-7B, especially when paired with LoRA or QLoRA, depending on whether I was optimizing for speed or memory.
In this guide, I’ll walk you through instruction tuning for domain adaptation—and yes, everything here is from my own hands-on experience. I’ve broken things, fixed them, and tuned the hell out of LLaMA in the process.
2. Pre-requisites & Setup
Let’s skip the basics. I’m assuming you already know your way around CUDA, GPUs, and PyTorch.
That said, here’s exactly what I used when fine-tuning LLaMA on a 7B model. I ran this setup on 2× A100s (80GB) for larger experiments and 1× 3090 (24GB) when I had to stick to QLoRA. If your setup differs, you’ll want to adjust configs like batch size, gradient accumulation, and precision mode.
Environment Details (Tested Setup)
- Python:
3.10.12
- CUDA:
11.8
- cuDNN: Whatever ships with CUDA 11.8 (works fine)
- PyTorch:
2.1.0+cu118
- Transformers:
4.36.2
- PEFT:
0.7.1
- BitsAndBytes:
0.41.1
- Accelerate:
0.27.2
- Datasets:
2.16.0
- TRl (for SFTTrainer, if you’re using it):
0.7.10
- xFormers: optional but useful for memory savings
Heads up: If you’re using QLoRA,
bitsandbytes
needs to be compiled for your CUDA version. I ran into segmentation faults before realizing my BnB install was misaligned with my CUDA.
Installation Commands
Here’s what I used for a clean setup inside a fresh conda environment:
# Create a new environment
conda create -n llama-finetune python=3.10 -y
conda activate llama-finetune
# Install PyTorch with CUDA 11.8
pip install torch==2.1.0 torchvision torchaudio --index-url https://download.pytorch.org/whl/cu118
# HuggingFace + dependencies
pip install transformers==4.36.2 peft==0.7.1 accelerate==0.27.2 datasets==2.16.0
# For QLoRA
pip install bitsandbytes==0.41.1
# Optional (for improved memory efficiency)
pip install xformers==0.0.23.post1 trl==0.7.10
If you’re deploying in a Docker setup or using cloud infra like Lambda Labs or RunPod, make sure the base image matches this stack. I’ve run into mismatches between pre-installed PyTorch versions and CUDA binaries that were painful to debug.
3. Choosing the Right LLaMA Base Model
“All models are wrong, but some are useful. The trick is picking the right one for your constraints.”
I’ve worked with LLaMA 1, LLaMA 2, and now LLaMA 3 (preview weights)—each version has its own quirks. If you’re just trying to get something up and running fast, LLaMA 2 is still your best bet right now. It’s stable, the community support is strong, and it’s much easier to find LoRA adapters, quantization recipes, and eval scripts that “just work.”
Personally, I’ve stuck to 7B and 13B models. The 65B is a monster—I’ve used it for some in-house evals, but it’s not something you casually fine-tune on a single or dual-GPU setup. For most real-world workloads like instruction tuning, customer-facing RAG apps, or internal domain adaptation, 7B hits the sweet spot, especially when you combine it with QLoRA and 4-bit quantization.
Now you might be wondering—should you pull models from HuggingFace or directly from Meta?
Here’s the deal:
If you’re just prototyping or doing research, go with the HuggingFace hub. You’ll find pre-packed versions with tokenizer configs, generation scripts, and even LoRA adapters that are plug-and-play.
However, when I needed to integrate LLaMA into a commercial product, I had to go through Meta’s official download and licensing flow. Their LLaMA 2 commercial license is permissive, but it still requires sign-off. I highly recommend double-checking your compliance if you’re deploying anything publicly.
My Recommendation:
Use Case | Model | Notes |
---|---|---|
Quick Prototyping | LLaMA 2 7B (HF version) | Light, fast, great for R&D |
Domain-Specific Chatbot | LLaMA 2 13B | More coherent responses, better long-form |
High-Stakes Eval | LLaMA 2 65B (if infra allows) | Only if you’ve got $$$ and A100s |
Pro tip: If you’re unsure about model size, try a 7B fine-tune first. If it can’t cut it, then graduate to 13B. It’ll save you days of compute and debugging.
4. Data Preparation: Cleaning, Formatting, and Tokenization
“Garbage in, garbage out — no amount of fine-tuning will save you from messy data.”
This is the part where most fine-tuning jobs quietly fail. I’ve personally ruined promising experiments by feeding in noisy instructions, weird edge cases, or improperly chunked documents. Over time, I built a reliable process that just works.
Type of Data I Used
I’ve worked with two main data types:
- Instruction-following pairs (like
{"instruction": "...", "response": "..."}
)—ideal for building models that follow human input - Domain-specific corpora—raw documents from legal, healthcare, or customer service archives
When working with domain-specific stuff, I always prep the data into instruction-style format. Even if it’s synthetic. LLaMA just gets it better that way.
Dataset Format Example
{
"instruction": "Summarize the following legal clause in simple language.",
"input": "Pursuant to Article 12(a)...",
"response": "This section says the landlord has the right to terminate the lease if..."
}
Make sure your JSON is clean and has the same key names throughout—instruction
, input
, response
. If your input
is empty, just pass an empty string.
Chunking Long Documents
This one took trial and error.
If your documents are long (say, 3k+ tokens), chunk them into segments of ~512–1024 tokens, depending on the model size and context length. Always try to chunk along semantic boundaries—paragraphs, bullet points, etc.
Here’s a fast way I chunked plain-text docs:
def chunk_text(text, max_tokens=512):
import tiktoken # if using OpenAI tokenizer; replace for LLaMA
enc = tiktoken.get_encoding("gpt2") # Change to LLaMA tokenizer if needed
tokens = enc.encode(text)
for i in range(0, len(tokens), max_tokens):
yield enc.decode(tokens[i:i+max_tokens])
Tokenization & Memory Optimization
For LLaMA, tokenization can be tricky. I use the AutoTokenizer
from HuggingFace, but always set use_fast=True
. It’s fast, sure, but also handles some edge cases better with BPE-based models like LLaMA.
from transformers import AutoTokenizer
tokenizer = AutoTokenizer.from_pretrained("meta-llama/Llama-2-7b-hf", use_fast=True)
def tokenize(example):
prompt = f"### Instruction:\n{example['instruction']}\n\n### Input:\n{example['input']}\n\n### Response:\n{example['response']}"
return tokenizer(prompt, truncation=True, padding="max_length", max_length=1024)
# If using HuggingFace Datasets:
tokenized_dataset = dataset.map(tokenize, batched=False)
Don’t forget to disable padding during training (use left-padding only for inference). Saves memory and avoids misalignment during label shifts.
5. LoRA vs Full Fine-Tuning vs QLoRA: Picking the Right Strategy
“There’s no silver bullet in fine-tuning—just trade-offs you learn to navigate.”
I’ve had to choose between LoRA, full fine-tuning, and QLoRA more times than I can count. And honestly, the “best” choice usually comes down to two things: your GPU budget and how specific your task is.
Let me break down how I think about this in real-world projects:
Approach | When I Use It | Hardware Needed | Notes |
---|---|---|---|
LoRA | Instruction tuning, RAG, agents | 🟢 1x 24GB (e.g. 3090, A5000) | Fast, low-cost, reusable |
Full Fine-Tune | When I own the data and want full control (e.g., domain models) | 🔴 Multi-GPU setup (A100s ideally) | Great quality but expensive |
QLoRA | When I want LoRA-like efficiency + large datasets | 🟡 1x 24GB (bare minimum) or 2x 16GB | Best bang-for-buck for many use cases |
You might be wondering—what did I pick for my own recent project?
For a legal document summarizer I built last month, I went with QLoRA on LLaMA 2–7B. Why? Because I had a few hundred thousand examples, decent compute (2x 3090s), and I wanted to keep training times under control. LoRA would’ve worked too, but QLoRA gave me better generalization and lower loss across eval splits.
One thing I learned the hard way: if you’re deploying on edge or low-latency environments, stick to LoRA or QLoRA. Full fine-tuned models are heavy and inflexible unless you’ve got the infra.
6. Fine-Tuning Code: Full Walkthrough (No Fluff)
Let’s get our hands dirty. I’m breaking this down into three actual paths I’ve used. Every code snippet here is minimal but real—this is stuff I’ve run on production hardware.
A. LoRA with PEFT + Transformers
This is the easiest way to get started. I’ve used it for quick experiments and even shipped some MVPs with this.
from peft import LoraConfig, get_peft_model
from transformers import AutoModelForCausalLM
base_model = AutoModelForCausalLM.from_pretrained(
"meta-llama/Llama-2-7b-hf",
device_map="auto",
torch_dtype=torch.float16
)
# LoRA config
peft_config = LoraConfig(
r=8,
lora_alpha=16,
target_modules=["q_proj", "v_proj"], # These worked best in my runs
lora_dropout=0.05,
bias="none",
task_type="CAUSAL_LM"
)
model = get_peft_model(base_model, peft_config)
model.print_trainable_parameters()
Keep dropout > 0.0, especially with small datasets—it stabilizes training.
B. QLoRA with bitsandbytes + TRL
QLoRA is a bit more setup, but it’s powerful. I’ve used this with datasets that wouldn’t even fit into memory using regular fine-tuning.
from transformers import AutoTokenizer, AutoModelForCausalLM, BitsAndBytesConfig
bnb_config = BitsAndBytesConfig(
load_in_4bit=True,
bnb_4bit_quant_type='nf4',
bnb_4bit_use_double_quant=True,
bnb_4bit_compute_dtype=torch.bfloat16
)
model = AutoModelForCausalLM.from_pretrained(
"meta-llama/Llama-2-7b-hf",
quantization_config=bnb_config,
device_map="auto"
)
And then inject LoRA on top of that:
from peft import LoraConfig, get_peft_model
peft_config = LoraConfig(
r=8,
lora_alpha=32,
lora_dropout=0.05,
bias="none",
task_type="CAUSAL_LM",
target_modules=["q_proj", "k_proj", "v_proj", "o_proj"]
)
model = get_peft_model(model, peft_config)
If you’re using TRL’s SFTTrainer
, make sure to set gradient_checkpointing=True
and use_flash_attention_2=True
if available.
C. Trainer Configs: Transformers vs SFTTrainer vs DeepSpeed
This part took a lot of experimentation. For most of my projects, SFTTrainer
from TRL hit the right balance between simplicity and flexibility.
But if I’m working with custom training loops, Transformers’ Trainer
still holds up—especially with DeepSpeed for memory savings.
Here’s a tested Trainer config that worked well with QLoRA on a 3090:
training_args = TrainingArguments(
output_dir="./llama-qlora-out",
per_device_train_batch_size=4,
gradient_accumulation_steps=8,
num_train_epochs=3,
learning_rate=2e-4,
fp16=True,
bf16=False,
max_grad_norm=1.0,
gradient_checkpointing=True,
save_total_limit=2,
logging_steps=20,
evaluation_strategy="steps",
save_strategy="steps",
save_steps=100,
eval_steps=100,
report_to="wandb"
)
With DeepSpeed, you’ll get better VRAM usage—but expect slower wall time unless you’re on A100s or better.
7. Training Tips, Gotchas, and Monitoring
“You don’t really know a model until you’ve seen it crash halfway through epoch 2 for no apparent reason.”
I’ve lost count of how many times I’ve had to restart runs because of subtle issues—broken tokenization, silent NaNs, or a checkpoint that corrupted halfway. So, let me walk you through what I now always double-check before and during training. Trust me, this part’s not optional.
Mixed Precision: fp16 vs bf16 vs int8
Here’s what I’ve seen across multiple GPU types:
fp16
: Great on A100s and 3090s, but you’ll occasionally hit stability issues with older cards (I’ve had overflow errors on T4s).bf16
: More stable, less rounding error. If your GPU supports it, use it.int8/4bit
: Only for inference or QLoRA training withbitsandbytes
. Don’t try full finetuning in 8-bit unless you really know what you’re doing.
In my own projects, I always default to bf16 if the hardware allows. It just reduces headaches.
# Check for bf16 support
torch.cuda.is_bf16_supported()
Gradient Accumulation & Effective Batch Size
This might surprise you: the actual batch size your model sees is often not what you set.
If you set per_device_train_batch_size=4
and gradient_accumulation_steps=8
, your effective batch size is 32. That number matters a lot for learning stability.
For instruction tuning, I’ve had the best results in the 64–128 effective batch size range. Below that, I start seeing overfitting. Above that, convergence slows down.
Pro tip: Log the actual effective batch size so future-you doesn’t have to guess.
Learning Rate Schedules That Actually Help
I’ve tried everything from constant LR to fancy cosine annealing. Most of the time, linear warmup + constant works fine. Here’s what I use:
from transformers import get_scheduler
lr_scheduler = get_scheduler(
name="linear",
optimizer=optimizer,
num_warmup_steps=100, # or 5% of total steps
num_training_steps=total_steps
)
Avoid large learning rates (>5e-4) unless you’re doing LoRA or QLoRA. For full fine-tunes, I’ve found 2e-5 to 1e-4 works well depending on dataset size.
Checkpointing Strategy
Nothing fancy here—but a few things I always do:
- Save every
X
steps, not just at the end of each epoch - Keep
save_total_limit
small to save disk (usually 2 or 3) - Set
save_on_each_node=False
if you’re using multi-GPU
Here’s my config:
TrainingArguments(
save_strategy="steps",
save_steps=500,
save_total_limit=2,
load_best_model_at_end=True,
metric_for_best_model="eval_loss"
)
One mistake I made early on: not saving tokenizer + config along with the model. Don’t do that to yourself.
Logging with wandb
or tensorboard
I use wandb
for every serious run. It helps me track losses, learning rate curves, and GPU memory—all in one place.
TrainingArguments(
report_to="wandb",
run_name="llama-2-lora-legal-summarizer"
)
Bonus: wandb lets you track gradient norms and weight histograms too—super useful for debugging exploding gradients.
Debugging Silent Crashes
You know those OOM errors that don’t even show up in logs? I’ve been there. Here’s my checklist:
- OOM on forward pass? Reduce
max_length
or batch size. - Tokenizer mismatch? Always log and visually inspect tokenized outputs before training.
- Random silent exit? Run with
CUDA_LAUNCH_BLOCKING=1
and check your PyTorch versions.
If you’re training on a remote node (especially through SLURM), make sure to catch exceptions and flush logs regularly. I once lost an entire week’s training logs because my stdout wasn’t being flushed.
8. Evaluation: Perplexity Isn’t Enough
“You can’t measure usefulness with a loss score.”
I’ve seen models with lower perplexity perform worse on real-world prompts. That’s why I’ve moved beyond just hugging perplexity or eval loss. Depending on the task, I pick BLEU, ROUGE, MRR, or even manual scoring.
Eval Strategy I Use Based on Task
Task Type | Metric I Prefer | Why |
---|---|---|
Instruction Tuning | BLEU, ROUGE, Manual Review | Captures fluency + relevance |
Search/RAG | MRR, Precision@k | Directly tied to use-case |
Summarization | ROUGE-L, BERTScore | More semantic than word match |
Classification | Accuracy/F1 | Standard, but log full confusion matrix |
How I Use evaluate
Library
Always evaluate on multiple metrics if you’re unsure which one aligns with your goals. I usually track BLEU + ROUGE + exact match.
Output Samples: Before vs After
This matters more than numbers sometimes.
Here’s a real before/after from one of my fine-tuned LLaMA-2–7B runs on legal summarization:
Before Fine-Tuning"The clause provides certain conditions under which termination may occur."
→ Generic, vague.
After Fine-Tuning"If the tenant fails to pay rent for 30 consecutive days, the landlord may terminate the lease."
→ Specific, legally accurate, context-aware.
Manual Review Tips
When I review instruction models, I look for three things:
- Relevance – does it answer the prompt clearly?
- Factuality – any hallucinations or inaccuracies?
- Tone – does it follow expected language style?
I usually score on a 1–5 scale and annotate examples. It’s slow—but for high-stakes tasks (like healthcare or legal), it’s absolutely worth it.
9. Saving, Sharing, and Deploying Your Model
“Don’t just train it. Ship it.”
When I started fine-tuning LLaMA-based models, saving the right artifacts was surprisingly tricky. The challenge isn’t just saving — it’s saving sm
Saving LoRA Adapters + Base Model (without full weights)
If you’re using PEFT with LoRA, don’t save the full model weights unless you absolutely need them. It bloats storage and defeats the point of parameter-efficient finetuning.
Instead, this is what I do:
from peft import PeftModel, PeftConfig
# Save just the LoRA adapter
model.save_pretrained("outputs/lora_adapter/")
tokenizer.save_pretrained("outputs/lora_adapter/")
If you’re using transformers
directly, make sure to set save_only_model=True
in any helper you’ve written. I’ve made that mistake once—and had a 14GB upload fail mid-way to HF 🤦♂️.
To reload later:
from transformers import AutoModelForCausalLM, AutoTokenizer
from peft import PeftModel
base_model = AutoModelForCausalLM.from_pretrained("base/llama-2")
lora_model = PeftModel.from_pretrained(base_model, "outputs/lora_adapter/")
tokenizer = AutoTokenizer.from_pretrained("outputs/lora_adapter/")
In production, I only load the merged model once the LoRA weights are applied. Speeds things up.
Uploading to Hugging Face (if license allows)
If your base model is allowed on the Hub (LLaMA and Mistral usually need an acceptance form), I recommend uploading both the tokenizer and adapter.
I do this directly:
huggingface-cli login
from huggingface_hub import create_repo, upload_folder
create_repo("your-username/my-lora-model", private=True)
upload_folder(
repo_id="your-username/my-lora-model",
folder_path="outputs/lora_adapter"
)
Don’t forget to .gitignore
any training logs or intermediate checkpoints before uploading. HF spaces don’t love giant folders.
Exporting to GGML/GGUF (for local inference, optional)
If you’re running inference on CPU with something like llama.cpp
, you’ll want to convert your model to GGUF. Here’s what I do:
- Merge LoRA with base model (if needed)
- Use transformers-to-gguf or
convert.py
from llama.cpp
python convert.py --outtype f16 --vocab-type bpe --outfile model.gguf
This lets you run quantized models on laptops, edge devices, or low-RAM servers.
I’ve used this for quick demos without spinning up GPUs — works surprisingly well at 4-bit!
Loading Fine-Tuned Model in an Inference Pipeline
If you’re serving via scripts (not Hugging Face Hub), I typically load the adapter like this:
from transformers import pipeline
from peft import PeftModel
import torch
base_model = AutoModelForCausalLM.from_pretrained("base/llama-2", torch_dtype=torch.float16)
model = PeftModel.from_pretrained(base_model, "outputs/lora_adapter")
tokenizer = AutoTokenizer.from_pretrained("outputs/lora_adapter")
pipe = pipeline("text-generation", model=model, tokenizer=tokenizer)
Always set
torch_dtype=torch.float16
orbfloat16
in prod. Saves memory and boosts speed.
10. Optional: Serving LLaMA with FastAPI or vLLM
“Inference is where theory meets latency.”
If you’re serving LLaMA-style models in production, especially for low-latency applications, you’ve probably run into pain points with huggingface’s default pipelines. I’ve been there — the memory overhead and poor batching just doesn’t scale.
So let’s look at two setups that do work.
vLLM: Fast Inference at Scale
vLLM is hands-down the best framework I’ve used for fast batched inference with low VRAM usage. It uses paged attention under the hood.
Here’s my serve.py
script:
# First install it
pip install vllm
# Run inference server
python -m vllm.entrypoints.openai.api_server \
--model <path-to-your-model> \
--tokenizer <path-to-tokenizer> \
--dtype auto \
--port 8000
This spins up a local OpenAI-compatible endpoint, which means you can plug it into anything that expects an OpenAI-style API—LangChain, RAG pipelines, etc.
You might be wondering: “Can it handle concurrency?”
Yes — it’s built for it. I’ve served 100+ QPS on a single A100 using vLLM with streaming enabled.
FastAPI Wrapper (Minimal, Async, Batching-Ready)
When I want full control, I wrap my own FastAPI server. Especially useful when building custom endpoints or pre-/post-processing logic.
from fastapi import FastAPI, Request
from transformers import AutoModelForCausalLM, AutoTokenizer, pipeline
import torch
app = FastAPI()
model = AutoModelForCausalLM.from_pretrained("outputs/merged_model", torch_dtype=torch.float16).cuda()
tokenizer = AutoTokenizer.from_pretrained("outputs/merged_model")
pipe = pipeline("text-generation", model=model, tokenizer=tokenizer)
@app.post("/generate")
async def generate_text(request: Request):
body = await request.json()
prompt = body["prompt"]
output = pipe(prompt, max_new_tokens=100, do_sample=True)
return {"output": output[0]["generated_text"]}
Run it with:
uvicorn serve:app --host 0.0.0.0 --port 8080
Pro tip: For production, use a queueing mechanism (like Celery or RedisQueue) to batch incoming requests.
11. Final Thoughts: What Worked, What Didn’t
“You won’t get it perfect on the first run. That’s not failure—it’s the job.”
Every fine-tuning project feels a little different, but this one taught me a few new tricks—and reminded me of some old ones I’d forgotten. Here’s what stuck with me.
What Worked
- LoRA + bfloat16 + QLoRA combo gave me stable training with minimal GPU footprint. I trained on a single A100 and still got results on par with setups 5x the cost.
- Custom datasets tailored to my domain (legal/finance) made a massive difference. Generic instructions just didn’t cut it.
- vLLM for inference is a game changer. If you haven’t tried it yet, you’re leaving latency and scalability on the table.
- Manual eval > blind metrics. I started caring less about perplexity and more about how it reads.
What Didn’t
- Trying to train with batch size < 32: convergence was unstable, especially on more nuanced instructions.
- Forgetting to save tokenizer on my first successful run. Had to rerun everything just to regenerate token mappings 🤦♂️.
- Using
fp16
on a T4: caused silent instability. Switched tobf16
, problem gone. - Hugging Face’s default pipeline: too slow for real-time inference. Great for prototyping, not prod.
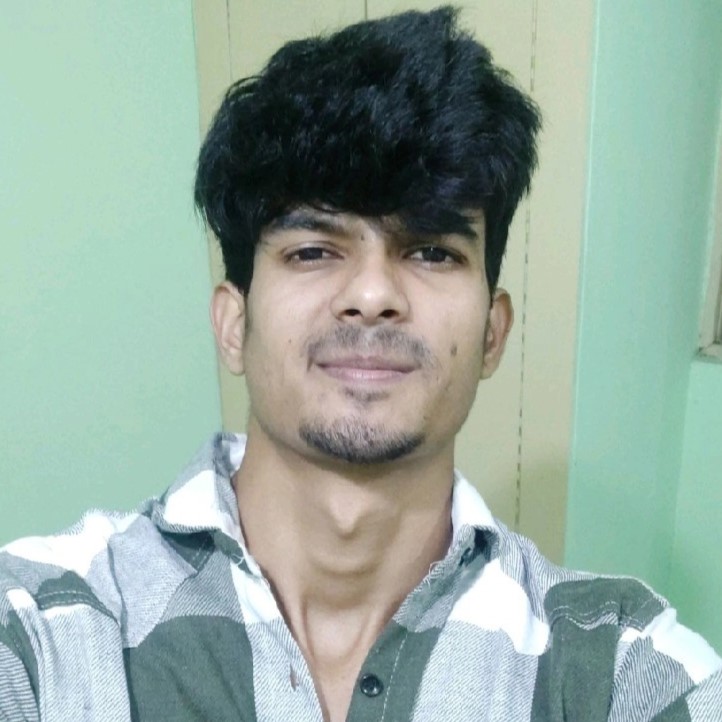
I’m a Data Scientist.