1. Introduction
“Give me a tool that can think and act for me—and hook it up to everything I use daily. That’s the dream.”
When I started experimenting with building AI agents that could actually do things, not just chat or generate text, I ran into two recurring problems:
- Orchestrating logic-heavy flows between different LLM components was a mess.
- Connecting those flows to external tools like Google Drive, Slack, or Notion meant hours of manual API wrangling.
That’s where Langflow and Composio made a huge difference in my workflow. Langflow lets me visually design and manage LLM pipelines—without getting buried in LangChain boilerplate. Composio, on the other hand, gives me plug-and-play access to third-party tools I use every day.
In this guide, I’ll walk you through exactly how I built a simple but powerful AI agent using both tools. For context, the agent fetches files from a shared Google Drive folder, summarizes them using GPT-4, and sends a digest to Slack.
This isn’t a LangChain 101. You won’t find abstract theory here. If you’re already deep into AI tooling and want a practical build that just works, you’re in the right place.
2. Stack Overview (TL;DR for the Impatient Pros)
Let’s quickly lay out what you’ll be working with. Here’s the minimal but powerful stack I used to pull this off:
Tool | Role in the System | Notes |
---|---|---|
Langflow | Visual builder for LangChain agents | Great for debugging and modular design |
Composio | OAuth-ready toolkit for third-party app actions | Think Zapier, but built for AI workflows |
LLM (GPT-4) | Core reasoning + summarization engine | You can swap this with a local LLM if needed |
Backend (optional) | FastAPI wrapper to expose as a service | Only if you want to make it callable |
Data Layer (optional) | FAISS / Chroma for search or context retrieval | Skip it if your agent doesn’t need memory |
You don’t have to use a vector store unless your use case requires long-term memory or search across large corpora. In my case, I skipped it because the agent was stateless and task-specific.
Here’s a high-level architecture I used for reference:
[User Input]
↓
[Langflow Chain]
↓
[Composio Tool Call → Google Drive]
↓
[LLM → Summarize]
↓
[Composio Tool Call → Slack Message]
It’s clean, scalable, and fast to iterate on. No tangled Python scripts or auth nightmares.
3. Set Up Your Environment
“Before you build something smart, you’ve got to set it up smart.”
This is the part where most people mess around for hours trying to get Langflow and Composio to play nice. I’ve been there. Trust me—if you don’t nail your setup right from the start, you’ll end up debugging weird issues that have nothing to do with your actual agent logic.
Here’s how I set up my environment efficiently, after some painful trial and error.
3.1 Langflow Installation (with real-world gotchas)
Option 1: Docker
Personally, I lean toward Docker for most Langflow projects—especially when I’m working across multiple environments or collaborating with teammates.
Here’s a Docker setup I’ve used that keeps things clean and reproducible:
git clone https://github.com/logspace-ai/langflow
cd langflow
docker-compose up --build
Why Docker?
- No version mismatch headaches
- Isolated from your local Python environment
- Easier to ship and deploy later
But here’s the catch: custom components (like tool wrappers or prompt formatters) require you to mount them properly inside the container. I once spent a full hour wondering why my custom Python file wasn’t showing up—turns out it wasn’t even being mounted.
If you’re using Docker, make sure you bind your local folder like this in docker-compose.yml
:
volumes:
- ./components:/app/langflow/components
Option 2: pip (for quick iteration)
If you’re just exploring or doing local testing, pip is faster:
pip install langflow
langflow run
The downside? You’ll eventually run into dependency hell—especially if you’re adding other LangChain components. I’ve had conflicts with openai
, chromadb
, and pydantic
versions. So unless I’m prototyping something tiny, I stick with Docker.
Version Tip
Langflow moves fast, and so does LangChain. Pin your versions. Here’s what worked for me recently:
langflow==0.1.45
langchain==0.1.14
Otherwise, you’ll randomly find that components disappear or chains start breaking silently after an update. (Been there. Not fun.)
3.2 Composio Integration
Here’s where the magic happens. Composio acts like a smart assistant that speaks the API dialects of every tool you need—Google Drive, Notion, Slack, and so on.
Step 1: Get Your API Key
Head to Composio’s dashboard and sign up. Once you’re in, grab your personal access token (PAT). This token is your master key to interact with any service through Composio.
I usually store mine in .env
:
COMPOSIO_API_KEY=sk-xyz-your-key-here
Never hardcode this in your scripts or Langflow blocks—especially if you’re deploying later.
Step 2: Install the SDK
Even though Langflow abstracts most of it, I still install the Composio SDK so I can test things programmatically before plugging them into the agent.
pip install composio-sdk
Here’s a quick sanity check to verify it’s working:
from composio_sdk import Composio
client = Composio(api_key="sk-xyz-your-key-here")
print(client.list_apps())
If you see a list of integrations like ["google_drive", "slack", "notion"]
, you’re good to go.
Step 3: Add Composio to Langflow
Now open Langflow, drag in the Tool block, and select ComposioTool.
You’ll need to:
- Paste in your API key
- Authorize any services you plan to use (OAuth flow will open in browser)
- Make sure scopes match the actions you plan to call (I once couldn’t delete a file from Google Drive because I had read-only access 😅)
Step 4: Security Notes
This might seem obvious, but it’s worth repeating—treat your Composio key like a root password. Don’t commit it to Git, and definitely don’t expose it in front-end code if you build a web wrapper later.
If you’re deploying, I recommend using secrets managers (e.g., Render, Vercel, or AWS Secrets Manager). I personally use .env
with dotenv
during development and mount secrets into containers in production.
4. Designing the Agent Flow in Langflow
“A good agent doesn’t just know what to do—it knows when, how, and why to do it.”
I’ve found that the design phase in Langflow is where everything either clicks or completely unravels. Once I started thinking in terms of chains instead of scripts, my workflows became way more maintainable and way less brittle.
This section is the meat of the whole thing—let’s break it down step-by-step, using a real use case I built myself.
4.1 Define the Agent’s Goal
Here’s what I wanted this agent to do for me:
“Search Google Drive for files containing a specific keyword in the title, summarize their content with GPT-4, and send a digest to Slack.”
Nothing fancy, but very useful in my day-to-day workflow. I use it to keep tabs on meeting notes dropped by different team members, without having to open each doc manually.
4.2 Build the Langflow Chain
Once I had the goal clear, I sketched the Langflow chain like this:
[Input] → [Prompt Template] → [Tool Selector] → [Composio Tool] → [Output]
Let’s walk through what each block does and what you should watch for when wiring it up.
Input Block
Start with a simple input block where the user can provide the keyword—this is what your agent will search for in Google Drive file titles.
Prompt Template
Now, here’s where I’ve learned that prompt engineering really matters—especially when you’re chaining tools. You need a well-structured template that:
- Clearly sets GPT’s role (summarizer, not analyzer)
- Instructs it on expected format (e.g., bullet points, short paragraphs)
- Keeps output length within Slack’s message limit
Here’s a basic example I used inside the Prompt block:
You are an assistant that summarizes documents in plain language.
Given the content of a document below, summarize it in 3 bullet points.
{{file_content}}
Simple, but it keeps things consistent and digestible.
Tool Selector → Composio Tool
You might be wondering: “Why not connect Composio directly?”
Well, here’s the deal—Langflow lets you add conditional logic via the Tool Selector block. That gives your agent more flexibility later.
In my case, I hardcoded the Composio Google Drive tool call, but I’ve also built dynamic selectors that choose between Slack, Notion, or even email based on the context.
Once you drop in the Composio Tool, you’ll configure it to:
- Authenticate Google Drive (via the credentials you added earlier)
- Use the
search_files
andget_file_content
actions - Pass the result to the LLM summarizer chain
Output Block
After GPT generates the summary, the Output block can either:
- Show it in the UI
- Pipe it to another Composio Tool (e.g.,
send_slack_message
)
I personally route it to Slack via a second Composio call. Here’s a sample payload block I configured:
{
"channel": "#team-digest",
"message": "{{summary_output}}"
}
I’ve also used format_message()
as a helper block in the middle, when I need better formatting before sending.
4.3 Add Custom Components (if needed)
Sometimes the built-in tools aren’t enough—especially if you want things like retries, logging, or post-processing.
Here’s a case from my own experience:
I needed to handle occasional Google API rate limits gracefully (this happened more than once). So I wrote a tiny retry wrapper around Composio’s SDK.
Here’s a stripped-down version of the custom tool:
from langchain.tools import BaseTool
from composio_sdk import Composio
import time
class ResilientDriveFetcher(BaseTool):
name = "resilient_drive_fetcher"
description = "Fetches Google Drive files with retry logic"
def __init__(self, api_key):
self.client = Composio(api_key=api_key)
def _run(self, query):
for _ in range(3):
try:
return self.client.call_tool(
app="google_drive",
tool="search_files",
input={"query": query}
)
except Exception as e:
time.sleep(2)
raise RuntimeError("All retries failed.")
Once written, I dropped this into my Langflow components/
directory and restarted the app. It shows up just like any other tool.
5. Wiring Up Composio Tools
“If Langflow is the brain, Composio is the nervous system—connecting your agent to the real world.”
This is where things get practical. And I mean really practical. I’ve personally burned a few hours here getting Composio to behave the way I needed—especially when chaining more than one tool or passing dynamic input. If you’ve worked with any middleware before, you’ll immediately recognize the power and pitfalls here.
5.1 Use Case-Specific Configuration
Let’s walk through a real example I set up recently:
Triggering a Slack message whenever a Google Calendar event with “demo” in the title is coming up within the next 60 minutes.
This might sound like a trivial integration, but here’s what tripped me up initially: Langflow’s inputs don’t always align neatly with what Composio expects.
First, Set Up the Composio Block
You’ll drop a Composio Tool block in Langflow and configure it like so:
{
"app": "google_calendar",
"tool": "list_events",
"input": {
"query": "demo",
"time_range": "next_1_hour"
}
}
Now here’s the thing most docs won’t tell you:
Composio actions often return nested JSON with inconsistent key formats (especially when events are returned from different sources or calendars).
So in Langflow, I added a custom transformer block (Python component) to sanitize the output before handing it off to the summarizer:
def clean_event_data(events):
return [
{
"title": e.get("summary"),
"time": e.get("start", {}).get("dateTime", "Unknown")
}
for e in events if "summary" in e
]
Mapping to Slack Message
Once you’ve got the cleaned-up list of events, pass it into the Slack block:
{
"app": "slack",
"tool": "send_message",
"input": {
"channel": "#demos",
"message": "{{formatted_event_summary}}"
}
}
I formatted the summary upstream, so Slack just acts as the output endpoint.
Error Handling Tips (From Painful Experience)
Now here’s the kicker—Composio doesn’t always throw clean error messages.
You might be wondering: “How do I catch API failures in Langflow?”
Well, I’ve personally wrapped most Composio calls in a try-catch-style custom tool, like this:
def run_composio_with_logging(app, tool, input_dict):
try:
return composio.call_tool(app=app, tool=tool, input=input_dict)
except Exception as e:
log_error(f"Composio error on {app}/{tool}: {str(e)}")
return {"error": str(e)}
Then, inside Langflow, I check for the "error"
key and branch accordingly using conditional logic blocks.
5.2 Chaining Multiple Composio Actions
Let’s step it up:
“Fetch recent meeting notes from Notion, summarize them, and post to Slack every day at 6 PM.”
I’ve built this exact flow and here’s how I structured it.
Step 1: Notion Fetch
Composio supports Notion’s list_blocks
and get_page_content
tools. The key is filtering pages based on a tag or title pattern.
Langflow config:
{
"app": "notion",
"tool": "search_pages",
"input": {
"query": "Meeting Notes",
"last_edited": "today"
}
}
Step 2: Intermediate Processing
I wrote a mid-pipeline block to:
- Deduplicate notes (if edited multiple times)
- Truncate any overly verbose pages
Here’s a tiny helper I used:
def truncate_notes(content, max_chars=1500):
return content[:max_chars] + "..." if len(content) > max_chars else content
Step 3: Slack Summary
After summarization, you drop the output to Slack—same as before, but you must pass the summary downstream using Langflow’s Output variable system ({{summary}}
).
Rate Limits and Latency (Yes, They Matter)
This might surprise you: Composio does throttle if you’re hammering APIs across multiple apps. Slack and Notion both have tight rate limits—you’ll get 429’d if you’re not pacing your calls.
Personally, I added a simple delay block before Slack to stagger sends in workflows that loop over multiple pages:
import time
time.sleep(1) # crude but effective
You could get fancier with asyncio, but honestly, for most Langflow agents, simplicity wins.
Wiring Composio tools might look plug-and-play at first glance, but once you start chaining and manipulating real-world data, that’s when the cracks show up. Hopefully, what I’ve shared here saves you the two evenings I lost chasing malformed payloads and silent failures.
6. Testing the Agent
“Code that isn’t tested is just a rumor.” — I live by this when working with Langflow agents.
Testing in Langflow isn’t just about seeing if something runs—it’s about understanding how your agent thinks. I’ve learned (the hard way) that GPT-based agents can pass a basic smoke test but still behave unpredictably under real conditions. Here’s how I personally test before shipping.
6.1 Input/Output Testing in Langflow
Start inside the Langflow canvas. Hit the “Run” button after wiring your flow—pretty standard stuff. But here’s what I focus on:
- Injecting realistic test inputs: I avoid generic queries like “summarize this”. Instead, I use inputs I expect from real users, like:
{
"query": "What did we discuss in the weekly marketing call about product launch timelines?"
}
- Watching how the agent routes logic: Especially if you’re using conditional flows or tool selectors, verify that the right path gets triggered based on your input.
6.2 Logs and Debugging Tips
This might surprise you: most of my debugging happens in the Langflow backend console, not the UI.
Here’s what I do:
🔹 Enable verbose logging:
Inside your Langflow .env
or config file:
LOG_LEVEL=DEBUG
This gives you internal logs when tools run, memory is updated, or a prompt fails to parse.
🔹 Check Composio responses:
Whenever a tool misbehaves, I inspect the raw JSON returned. Composio blocks in Langflow let you capture that:
# inside a debug block
print("Composio raw output:", output)
I’ve caught malformed payloads, expired tokens, and invalid field types this way.
6.3 Interpreting Agent Weirdness
You might be wondering: What if the agent gives a valid response… but not the one I expected?
That’s where prompt tuning and memory debugging come in.
My rule of thumb:
If the agent behaves inconsistently:
- First, check prompt context length (you might be overrunning the token window).
- Then, inspect whether memory history is polluting the context.
- Finally, log intermediate outputs—especially from the Prompt Template blocks.
Sometimes, I add a dummy echo
block just to surface variable values during flow execution. It’s saved me hours.
7. Deploying Your Agent
“If it only works on your machine, it’s not a product. It’s a prototype.”
Langflow makes local testing simple, but real-world usage means putting your agent somewhere accessible, reliable, and secure. Here’s how I’ve done it in production environments.
7.1 Local Deployment (for Testing)
I usually run Langflow locally during development using:
langflow run
You’ll get access at http://localhost:7860
.
Expose via Tunnel (ngrok)
To test webhooks or receive external inputs (e.g., from Slack or Composio callbacks), I use:
ngrok http 7860
Or with loca.lt:
npx localtunnel --port 7860
This lets you test Langflow integrations with live systems without deploying to a server.
7.2 Web App Interface (Optional)
If you’re like me and need a UI beyond Langflow’s visual builder—something your product managers or end-users can click—here’s what I use:
Streamlit Wrapper (Minimal UI)
import streamlit as st
from langflow import run_flow
user_input = st.text_input("Ask your agent:")
if st.button("Run"):
result = run_flow("your_flow.json", {"query": user_input})
st.write(result)
FastAPI (for APIs or app backends)
from fastapi import FastAPI
from langflow import run_flow
app = FastAPI()
@app.post("/run")
def run_agent(payload: dict):
return run_flow("your_flow.json", payload)
from fastapi import FastAPI
from langflow import run_flow
app = FastAPI()
@app.post(“/run”)
def run_agent(payload: dict):
return run_flow(“your_flow.json”, payload)
7.3 Deploy to Cloud (Optional Advanced)
This is where things get serious. I’ve used Render and Railway successfully, both of which handle Docker-based deployment well.
Dockerfile Example
FROM python:3.10
WORKDIR /app
COPY . /app
RUN pip install -r requirements.txt
EXPOSE 7860
CMD ["langflow", "run"]
Deploy that container using your cloud of choice. My go-to is Fly.io when I want fast deploys with free bandwidth.
Securing Composio Credentials
Do not hardcode your API keys.
I use environment variables:
export COMPOSIO_API_KEY="sk-..."
Or mount a .env
file with Docker:
docker run --env-file .env -p 7860:7860 my-langflow-agent
And in Langflow, I reference those variables using Jinja:
{
"api_key": "{{env.COMPOSIO_API_KEY}}"
}
That wraps up the core deployment flow. Once deployed, I usually set up a ping endpoint or simple uptime monitor to confirm the agent stays healthy.
8. Final Thoughts & Next Steps
I’ve built enough LangChain-based agents to know this: getting something to work is easy—making it robust and adaptable takes real thought.
Over the course of this build, you and I put together a working Langflow agent that connects to external tools using Composio, handles dynamic logic, and can be deployed to cloud environments. If you’ve followed along step-by-step, you now have an agent that’s not just functional—but extensible.
That last part is key. Because you’re not done. Not even close.
What You’ve Built
Let’s quickly recap:
- A Langflow-based GPT-4 agent that fetches and summarizes real data (e.g., Google Drive files).
- Integrated with Composio tools like Slack, Notion, and Google Calendar.
- Deployed and testable locally or in the cloud.
- Designed to be modular—with memory, tool selectors, and custom logic blocks.
This isn’t a toy project. With the right inputs, it’s something you could plug into a real org today.
Where to Take It Next
Here’s what I’ve personally added to similar agents in production—these are high-leverage improvements you might want to consider next:
Add a Vector Store
If you’re summarizing or answering based on large knowledge bases, plugging in a vector DB (like Pinecone or Chroma) makes a huge difference.
I usually embed with OpenAI or Cohere, then inject top-N chunks into the prompt template dynamically. You can do this with a custom LangChain Retriever and wire it right into Langflow.
Add More Tools
Composio supports a growing list of connectors—and once you’re comfortable chaining, adding these takes minutes:
- Zapier: Trigger workflows or push Langflow output into Zap automations.
- Airtable: Store extracted insights or summaries in structured rows.
- Salesforce: Update CRM fields directly from a conversation.
The nice thing? You can plug these in conditionally using a tool selector and let the agent decide what to call.
Schedule It
Agents don’t always need to wait for user input. I’ve set up scheduled runs to do things like:
- Pull calendar events, summarize meeting notes, and push summaries to Slack every morning.
- Check for new files in Google Drive hourly and update a team dashboard.
You can trigger Langflow flows via a small Python scheduler or use cron with a FastAPI wrapper. Keep it lightweight unless you need orchestration.
Where to Take It Next
Here’s what I’ve personally added to similar agents in production—these are high-leverage improvements you might want to consider next:
Add a Vector Store
If you’re summarizing or answering based on large knowledge bases, plugging in a vector DB (like Pinecone or Chroma) makes a huge difference.
I usually embed with OpenAI or Cohere, then inject top-N chunks into the prompt template dynamically. You can do this with a custom LangChain Retriever and wire it right into Langflow.
Add More Tools
Composio supports a growing list of connectors—and once you’re comfortable chaining, adding these takes minutes:
- Zapier: Trigger workflows or push Langflow output into Zap automations.
- Airtable: Store extracted insights or summaries in structured rows.
- Salesforce: Update CRM fields directly from a conversation.
The nice thing? You can plug these in conditionally using a tool selector and let the agent decide what to call.
Schedule It
Agents don’t always need to wait for user input. I’ve set up scheduled runs to do things like:
- Pull calendar events, summarize meeting notes, and push summaries to Slack every morning.
- Check for new files in Google Drive hourly and update a team dashboard.
You can trigger Langflow flows via a small Python scheduler or use cron with a FastAPI wrapper. Keep it lightweight unless you need orchestration.
If you’ve made it this far—seriously, great work. Most people stop at ChatGPT playgrounds. But what you’ve built is something that can scale.
When you’re ready, we can go deeper into topics like:
- Fine-tuning prompt engineering for multi-turn tools.
- Observability and agent telemetry (because when it breaks, logs matter).
- Orchestration with Prefect or Airflow for larger agent ecosystems.
Just let me know where you want to go next.
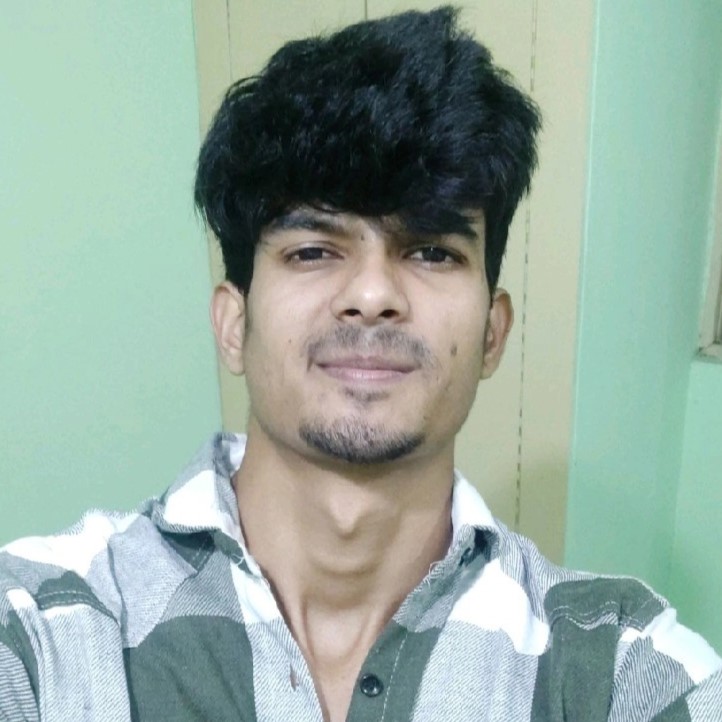
I’m a Data Scientist.